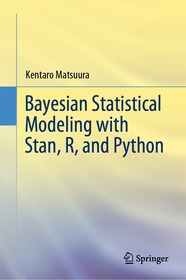
Bayesian Statistical Modeling with Stan, R, and Python
-
8% KEDVEZMÉNY?
- A kedvezmény csak az 'Értesítés a kedvenc témákról' hírlevelünk címzettjeinek rendeléseire érvényes.
- Kiadói listaár EUR 160.49
-
Az ár azért becsült, mert a rendelés pillanatában nem lehet pontosan tudni, hogy a beérkezéskor milyen lesz a forint árfolyama az adott termék eredeti devizájához képest. Ha a forint romlana, kissé többet, ha javulna, kissé kevesebbet kell majd fizetnie.
- Kedvezmény(ek) 8% (cc. 5 446 Ft off)
- Kedvezményes ár 62 633 Ft (59 650 Ft + 5% áfa)
Iratkozzon fel most és részesüljön kedvezőbb árainkból!
Feliratkozom
68 079 Ft
Beszerezhetőség
Becsült beszerzési idő: A Prosperónál jelenleg nincsen raktáron, de a kiadónál igen. Beszerzés kb. 3-5 hét..
A Prosperónál jelenleg nincsen raktáron.
Why don't you give exact delivery time?
A beszerzés időigényét az eddigi tapasztalatokra alapozva adjuk meg. Azért becsült, mert a terméket külföldről hozzuk be, így a kiadó kiszolgálásának pillanatnyi gyorsaságától is függ. A megadottnál gyorsabb és lassabb szállítás is elképzelhető, de mindent megteszünk, hogy Ön a lehető leghamarabb jusson hozzá a termékhez.
A termék adatai:
- Kiadás sorszáma 1st ed. 2022
- Kiadó Springer
- Megjelenés dátuma 2023. január 25.
- Kötetek száma 1 pieces, Book
- ISBN 9789811947544
- Kötéstípus Keménykötés
- Terjedelem385 oldal
- Méret 235x155 mm
- Súly 850 g
- Nyelv angol
- Illusztrációk 251 Illustrations, black & white; 10 Illustrations, color 457
Kategóriák
Rövid leírás:
This book provides a highly practical introduction to Bayesian statistical modeling with Stan, which has become the most popular probabilistic programming language.
The book is divided into four parts. The first part reviews the theoretical background of modeling and Bayesian inference and presents a modeling workflow that makes modeling more engineering than art. The second part discusses the use of Stan, CmdStanR, and CmdStanPy from the very beginning to basic regression analyses. The third part then introduces a number of probability distributions, nonlinear models, and hierarchical (multilevel) models, which are essential to mastering statistical modeling. It also describes a wide range of frequently used modeling techniques, such as censoring, outliers, missing data, speed-up, and parameter constraints, and discusses how to lead convergence of MCMC. Lastly, the fourth part examines advanced topics for real-world data: longitudinal data analysis, state space models, spatial data analysis, Gaussian processes, Bayesian optimization, dimensionality reduction, model selection, and information criteria, demonstrating that Stan can solve any one of these problems in as little as 30 lines.
Using numerous easy-to-understand examples, the book explains key concepts, which continue to be useful when using future versions of Stan and when using other statistical modeling tools. The examples do not require domain knowledge and can be generalized to many fields. The book presents full explanations of code and math formulas, enabling readers to extend models for their own problems. All the code and data are on GitHub.
Hosszú leírás:
This book provides a highly practical introduction to Bayesian statistical modeling with Stan, which has become the most popular probabilistic programming language.
The book is divided into four parts. The first part reviews the theoretical background of modeling and Bayesian inference and presents a modeling workflow that makes modeling more engineering than art. The second part discusses the use of Stan, CmdStanR, and CmdStanPy from the very beginning to basic regression analyses. The third part then introduces a number of probability distributions, nonlinear models, and hierarchical (multilevel) models, which are essential to mastering statistical modeling. It also describes a wide range of frequently used modeling techniques, such as censoring, outliers, missing data, speed-up, and parameter constraints, and discusses how to lead convergence of MCMC. Lastly, the fourth part examines advanced topics for real-world data: longitudinal data analysis, state space models, spatial data analysis, Gaussian processes, Bayesian optimization, dimensionality reduction, model selection, and information criteria, demonstrating that Stan can solve any one of these problems in as little as 30 lines.
Using numerous easy-to-understand examples, the book explains key concepts, which continue to be useful when using future versions of Stan and when using other statistical modeling tools. The examples do not require domain knowledge and can be generalized to many fields. The book presents full explanations of code and math formulas, enabling readers to extend models for their own problems. All the code and data are on GitHub.