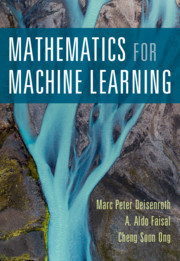
Mathematics for Machine Learning
-
10% KEDVEZMÉNY?
- A kedvezmény csak az 'Értesítés a kedvenc témákról' hírlevelünk címzettjeinek rendeléseire érvényes.
- Kiadói listaár GBP 39.99
-
Az ár azért becsült, mert a rendelés pillanatában nem lehet pontosan tudni, hogy a beérkezéskor milyen lesz a forint árfolyama az adott termék eredeti devizájához képest. Ha a forint romlana, kissé többet, ha javulna, kissé kevesebbet kell majd fizetnie.
- Kedvezmény(ek) 10% (cc. 2 024 Ft off)
- Discounted price 18 215 Ft (17 348 Ft + 5% áfa)
20 238 Ft
Beszerezhetőség
Becsült beszerzési idő: A Prosperónál jelenleg nincsen raktáron, de a kiadónál igen. Beszerzés kb. 3-5 hét..
A Prosperónál jelenleg nincsen raktáron.
Why don't you give exact delivery time?
A beszerzés időigényét az eddigi tapasztalatokra alapozva adjuk meg. Azért becsült, mert a terméket külföldről hozzuk be, így a kiadó kiszolgálásának pillanatnyi gyorsaságától is függ. A megadottnál gyorsabb és lassabb szállítás is elképzelhető, de mindent megteszünk, hogy Ön a lehető leghamarabb jusson hozzá a termékhez.
A termék adatai:
- Kiadó Cambridge University Press
- Megjelenés dátuma 2020. április 23.
- ISBN 9781108455145
- Kötéstípus Puhakötés
- Terjedelem398 oldal
- Méret 252x177x18 mm
- Súly 800 g
- Nyelv angol
- Illusztrációk 3 b/w illus. 106 colour illus. 2106
Kategóriák
Rövid leírás:
Distills key concepts from linear algebra, geometry, matrices, calculus, optimization, probability and statistics that are used in machine learning.
TöbbHosszú leírás:
The fundamental mathematical tools needed to understand machine learning include linear algebra, analytic geometry, matrix decompositions, vector calculus, optimization, probability and statistics. These topics are traditionally taught in disparate courses, making it hard for data science or computer science students, or professionals, to efficiently learn the mathematics. This self-contained textbook bridges the gap between mathematical and machine learning texts, introducing the mathematical concepts with a minimum of prerequisites. It uses these concepts to derive four central machine learning methods: linear regression, principal component analysis, Gaussian mixture models and support vector machines. For students&&&160;and others&&&160;with a mathematical background, these derivations provide a starting point to machine learning texts. For&&&160;those&&&160;learning the mathematics for the first time, the methods help build intuition and practical experience with applying mathematical concepts. Every chapter includes worked examples and exercises to test understanding. Programming tutorials are offered on the book's web site.
'This book provides great coverage of all the basic mathematical concepts for machine learning. I'm looking forward to sharing it with students, colleagues, and anyone interested in building a solid understanding of the fundamentals.' Joelle Pineau, McGill University, Montreal
Tartalomjegyzék:
1. Introduction and motivation; 2. Linear algebra; 3. Analytic geometry; 4. Matrix decompositions; 5. Vector calculus; 6. Probability and distribution; 7. Optimization; 8. When models meet data; 9. Linear regression; 10. Dimensionality reduction with principal component analysis; 11. Density estimation with Gaussian mixture models; 12. Classification with support vector machines.
Több