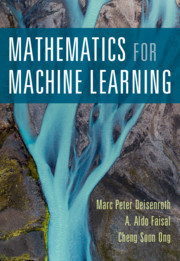
Mathematics for Machine Learning
Publisher: Cambridge University Press
Date of Publication: 23 April 2020
Normal price:
Publisher's listprice:
GBP 37.99
GBP 37.99
Your price:
16 514 (15 728 HUF + 5% VAT )
discount is: 10% (approx 1 835 HUF off)
The discount is only available for 'Alert of Favourite Topics' newsletter recipients.
Click here to subscribe.
Click here to subscribe.
Availability:
Estimated delivery time: In stock at the publisher, but not at Prospero's office. Delivery time approx. 3-5 weeks.
Not in stock at Prospero.
Can't you provide more accurate information?
Not in stock at Prospero.
Product details:
ISBN13: | 9781108455145 |
ISBN10: | 110845514X |
Binding: | Paperback |
No. of pages: | 398 pages |
Size: | 252x177x18 mm |
Weight: | 800 g |
Language: | English |
Illustrations: | 3 b/w illus. 106 colour illus. |
1126 |
Category:
Short description:
Distills key concepts from linear algebra, geometry, matrices, calculus, optimization, probability and statistics that are used in machine learning.
Long description:
The fundamental mathematical tools needed to understand machine learning include linear algebra, analytic geometry, matrix decompositions, vector calculus, optimization, probability and statistics. These topics are traditionally taught in disparate courses, making it hard for data science or computer science students, or professionals, to efficiently learn the mathematics. This self-contained textbook bridges the gap between mathematical and machine learning texts, introducing the mathematical concepts with a minimum of prerequisites. It uses these concepts to derive four central machine learning methods: linear regression, principal component analysis, Gaussian mixture models and support vector machines. For students&&&160;and others&&&160;with a mathematical background, these derivations provide a starting point to machine learning texts. For&&&160;those&&&160;learning the mathematics for the first time, the methods help build intuition and practical experience with applying mathematical concepts. Every chapter includes worked examples and exercises to test understanding. Programming tutorials are offered on the book's web site.
'This book provides great coverage of all the basic mathematical concepts for machine learning. I'm looking forward to sharing it with students, colleagues, and anyone interested in building a solid understanding of the fundamentals.' Joelle Pineau, McGill University, Montreal
'This book provides great coverage of all the basic mathematical concepts for machine learning. I'm looking forward to sharing it with students, colleagues, and anyone interested in building a solid understanding of the fundamentals.' Joelle Pineau, McGill University, Montreal
Table of Contents:
1. Introduction and motivation; 2. Linear algebra; 3. Analytic geometry; 4. Matrix decompositions; 5. Vector calculus; 6. Probability and distribution; 7. Optimization; 8. When models meet data; 9. Linear regression; 10. Dimensionality reduction with principal component analysis; 11. Density estimation with Gaussian mixture models; 12. Classification with support vector machines.