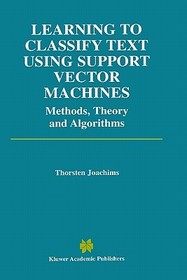
Learning to Classify Text Using Support Vector Machines
Methods, Theory and Algorithms
Sorozatcím: The Springer International Series in Engineering and Computer Science; 668;
-
20% KEDVEZMÉNY?
- A kedvezmény csak az 'Értesítés a kedvenc témákról' hírlevelünk címzettjeinek rendeléseire érvényes.
- Kiadói listaár EUR 106.99
-
Az ár azért becsült, mert a rendelés pillanatában nem lehet pontosan tudni, hogy a beérkezéskor milyen lesz a forint árfolyama az adott termék eredeti devizájához képest. Ha a forint romlana, kissé többet, ha javulna, kissé kevesebbet kell majd fizetnie.
- Kedvezmény(ek) 20% (cc. 9 077 Ft off)
- Kedvezményes ár 36 307 Ft (34 578 Ft + 5% áfa)
Iratkozzon fel most és részesüljön kedvezőbb árainkból!
Feliratkozom
45 385 Ft
Beszerezhetőség
Becsült beszerzési idő: A Prosperónál jelenleg nincsen raktáron, de a kiadónál igen. Beszerzés kb. 3-5 hét..
A Prosperónál jelenleg nincsen raktáron.
Why don't you give exact delivery time?
A beszerzés időigényét az eddigi tapasztalatokra alapozva adjuk meg. Azért becsült, mert a terméket külföldről hozzuk be, így a kiadó kiszolgálásának pillanatnyi gyorsaságától is függ. A megadottnál gyorsabb és lassabb szállítás is elképzelhető, de mindent megteszünk, hogy Ön a lehető leghamarabb jusson hozzá a termékhez.
A termék adatai:
- Kiadás sorszáma 2002
- Kiadó Springer
- Megjelenés dátuma 2002. április 30.
- Kötetek száma 1 pieces, Book
- ISBN 9780792376798
- Kötéstípus Keménykötés
- Terjedelem205 oldal
- Méret 235x155 mm
- Súly 1100 g
- Nyelv angol
- Illusztrációk XVII, 205 p. Illustrations, black & white 0
Kategóriák
Hosszú leírás:
Based on ideas from Support Vector Machines (SVMs), Learning To Classify Text Using Support Vector Machines presents a new approach to generating text classifiers from examples. The approach combines high performance and efficiency with theoretical understanding and improved robustness. In particular, it is highly effective without greedy heuristic components. The SVM approach is computationally efficient in training and classification, and it comes with a learning theory that can guide real-world applications.
Learning To Classify Text Using Support Vector Machines gives a complete and detailed description of the SVM approach to learning text classifiers, including training algorithms, transductive text classification, efficient performance estimation, and a statistical learning model of text classification. In addition, it includes an overview of the field of text classification, making it self-contained even for newcomers to the field. This book gives a concise introduction to SVMs for pattern recognition, and it includes a detailed description of how to formulate text-classification tasks for machine learning.
TöbbTartalomjegyzék:
1. Introduction.- 1 Challenges.- 2 Goals.- 3 Overview and Structure of the Argument.- 4 Summary.- 2. Text Classification.- 1 Learning Task.- 2 Representing Text.- 3 Feature Selection.- 4 Term Weighting.- 5 Conventional Learning Methods.- 6 Performance Measures.- 7 Experimental Setup.- 3. Support Vector Machines.- 1 Linear Hard-Margin SVMs.- 2 Soft-Margin SVMs.- 3 Non-Linear SVMs.- 4 Asymmetric Misclassification Cost.- 5 Other Maximum-Margin Methods.- 6 Further Work and Further Information.- Theory.- 4. A Statistical Learning Model of text Classification for SVMs.- 5. Efficient Performance Estimators for SVMs.- Methods.- 6. Inductive Text Classification.- 7. Transductive Text Classification.- Algorithms.- 8. Training Inductive Support Vector Machines.- 9. Training Transductive Support Vector Machines.- 10. Conclusions.- Appendices.- SVM-Light Commands and Options.
Több