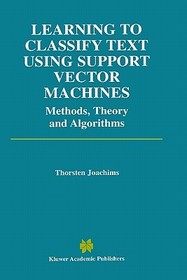
Learning to Classify Text Using Support Vector Machines
Methods, Theory and Algorithms
Series: The Springer International Series in Engineering and Computer Science; 668;
- Publisher's listprice EUR 106.99
-
The price is estimated because at the time of ordering we do not know what conversion rates will apply to HUF / product currency when the book arrives. In case HUF is weaker, the price increases slightly, in case HUF is stronger, the price goes lower slightly.
- Discount 8% (cc. 3 631 Ft off)
- Discounted price 41 753 Ft (39 765 Ft + 5% VAT)
45 385 Ft
Availability
Estimated delivery time: In stock at the publisher, but not at Prospero's office. Delivery time approx. 3-5 weeks.
Not in stock at Prospero.
Why don't you give exact delivery time?
Delivery time is estimated on our previous experiences. We give estimations only, because we order from outside Hungary, and the delivery time mainly depends on how quickly the publisher supplies the book. Faster or slower deliveries both happen, but we do our best to supply as quickly as possible.
Product details:
- Edition number 2002
- Publisher Springer
- Date of Publication 30 April 2002
- Number of Volumes 1 pieces, Book
- ISBN 9780792376798
- Binding Hardback
- No. of pages205 pages
- Size 235x155 mm
- Weight 1100 g
- Language English
- Illustrations XVII, 205 p. Illustrations, black & white 0
Categories
Long description:
Based on ideas from Support Vector Machines (SVMs), Learning To Classify Text Using Support Vector Machines presents a new approach to generating text classifiers from examples. The approach combines high performance and efficiency with theoretical understanding and improved robustness. In particular, it is highly effective without greedy heuristic components. The SVM approach is computationally efficient in training and classification, and it comes with a learning theory that can guide real-world applications.
Learning To Classify Text Using Support Vector Machines gives a complete and detailed description of the SVM approach to learning text classifiers, including training algorithms, transductive text classification, efficient performance estimation, and a statistical learning model of text classification. In addition, it includes an overview of the field of text classification, making it self-contained even for newcomers to the field. This book gives a concise introduction to SVMs for pattern recognition, and it includes a detailed description of how to formulate text-classification tasks for machine learning.
MoreTable of Contents:
1. Introduction.- 1 Challenges.- 2 Goals.- 3 Overview and Structure of the Argument.- 4 Summary.- 2. Text Classification.- 1 Learning Task.- 2 Representing Text.- 3 Feature Selection.- 4 Term Weighting.- 5 Conventional Learning Methods.- 6 Performance Measures.- 7 Experimental Setup.- 3. Support Vector Machines.- 1 Linear Hard-Margin SVMs.- 2 Soft-Margin SVMs.- 3 Non-Linear SVMs.- 4 Asymmetric Misclassification Cost.- 5 Other Maximum-Margin Methods.- 6 Further Work and Further Information.- Theory.- 4. A Statistical Learning Model of text Classification for SVMs.- 5. Efficient Performance Estimators for SVMs.- Methods.- 6. Inductive Text Classification.- 7. Transductive Text Classification.- Algorithms.- 8. Training Inductive Support Vector Machines.- 9. Training Transductive Support Vector Machines.- 10. Conclusions.- Appendices.- SVM-Light Commands and Options.
More