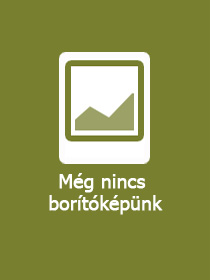
Evolutionary Multi-Task Optimization
Foundations and Methodologies
Sorozatcím: Machine Learning: Foundations, Methodologies, and Applications;
-
8% KEDVEZMÉNY?
- A kedvezmény csak az 'Értesítés a kedvenc témákról' hírlevelünk címzettjeinek rendeléseire érvényes.
- Kiadói listaár EUR 181.89
-
Az ár azért becsült, mert a rendelés pillanatában nem lehet pontosan tudni, hogy a beérkezéskor milyen lesz a forint árfolyama az adott termék eredeti devizájához képest. Ha a forint romlana, kissé többet, ha javulna, kissé kevesebbet kell majd fizetnie.
- Kedvezmény(ek) 8% (cc. 6 173 Ft off)
- Discounted price 70 985 Ft (67 604 Ft + 5% áfa)
77 157 Ft
Beszerezhetőség
Még nem jelent meg, de rendelhető. A megjelenéstől számított néhány héten belül megérkezik.
Why don't you give exact delivery time?
A beszerzés időigényét az eddigi tapasztalatokra alapozva adjuk meg. Azért becsült, mert a terméket külföldről hozzuk be, így a kiadó kiszolgálásának pillanatnyi gyorsaságától is függ. A megadottnál gyorsabb és lassabb szállítás is elképzelhető, de mindent megteszünk, hogy Ön a lehető leghamarabb jusson hozzá a termékhez.
A termék adatai:
- Kiadás sorszáma 1st ed. 2023
- Kiadó Springer
- Megjelenés dátuma 2025. április 2.
- Kötetek száma 1 pieces, Book
- ISBN 9789811956522
- Kötéstípus Puhakötés
- Terjedelem219 oldal
- Méret 235x155 mm
- Nyelv angol
- Illusztrációk 1 Illustrations, black & white 700
Kategóriák
Rövid leírás:
Recently, a novel evolutionary search paradigm, Evolutionary Multi-Task (EMT) optimization, has been proposed in the realm of evolutionary computation. In contrast to traditional evolutionary searches, which solve a single task in a single run, evolutionary multi-tasking algorithm conducts searches concurrently on multiple search spaces corresponding to different tasks or optimization problems,each possessing a unique function landscape. By exploiting the latent synergies among distinct problems, the superior search performance of EMT optimization in terms of solution quality and convergence speed has been demonstrated in a variety of continuous, discrete, and hybrid (mixture of continuous and discrete) tasks.
This book discusses the foundations and methodologies of developing evolutionary multi-tasking algorithms for complex optimization, including in domains characterized by factors such as multiple objectives of interest, high-dimensional search spaces and NP-hardness.
TöbbHosszú leírás:
Recently, a novel evolutionary search paradigm, Evolutionary Multi-Task (EMT) optimization, has been proposed in the realm of evolutionary computation. In contrast to traditional evolutionary searches, which solve a single task in a single run, evolutionary multi-tasking algorithm conducts searches concurrently on multiple search spaces corresponding to different tasks or optimization problems,each possessing a unique function landscape. By exploiting the latent synergies among distinct problems, the superior search performance of EMT optimization in terms of solution quality and convergence speed has been demonstrated in a variety of continuous, discrete, and hybrid (mixture of continuous and discrete) tasks.
This book discusses the foundations and methodologies of developing evolutionary multi-tasking algorithms for complex optimization, including in domains characterized by factors such as multiple objectives of interest, high-dimensional search spaces and NP-hardness.
TöbbTartalomjegyzék:
Chapter 1.Introduction.- Chapter 2. Overview and Application-driven Motivations of Evolutionary Multitasking.- Chapter 3.The Multi-factorial Evolutionary Algorithm.- Chapter 4. Multi-factorial Evolutionary Algorithm with Adaptive Knowledge Transfer.- Chapter 5.Explicit Evolutionary Multi-task Optimization Algorithm.- Chapter 6.Evolutionary Multi-task Optimization for Generalized Vehicle Routing Problem With Occasional Drivers.- Chapter 7. Explicit Evolutionary Multi-task Optimization for Capacitated Vehicle Routing Problem.- Chapter 8. Multi-Space Evolutionary Search for Large Scale Single-Objective Optimization.- Chapter 9.Multi-Space Evolutionary Search for Large-scale Multi-Objective Optimization.
Több