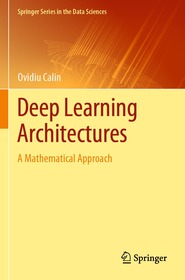
Deep Learning Architectures
A Mathematical Approach
Sorozatcím: Springer Series in the Data Sciences;
-
20% KEDVEZMÉNY?
- A kedvezmény csak az 'Értesítés a kedvenc témákról' hírlevelünk címzettjeinek rendeléseire érvényes.
- Kiadói listaár EUR 69.54
-
Az ár azért becsült, mert a rendelés pillanatában nem lehet pontosan tudni, hogy a beérkezéskor milyen lesz a forint árfolyama az adott termék eredeti devizájához képest. Ha a forint romlana, kissé többet, ha javulna, kissé kevesebbet kell majd fizetnie.
- Kedvezmény(ek) 20% (cc. 5 900 Ft off)
- Discounted price 23 599 Ft (22 475 Ft + 5% áfa)
Iratkozzon fel most és részesüljön kedvezőbb árainkból!
Feliratkozom
29 498 Ft
Beszerezhetőség
Becsült beszerzési idő: A Prosperónál jelenleg nincsen raktáron, de a kiadónál igen. Beszerzés kb. 3-5 hét..
A Prosperónál jelenleg nincsen raktáron.
Why don't you give exact delivery time?
A beszerzés időigényét az eddigi tapasztalatokra alapozva adjuk meg. Azért becsült, mert a terméket külföldről hozzuk be, így a kiadó kiszolgálásának pillanatnyi gyorsaságától is függ. A megadottnál gyorsabb és lassabb szállítás is elképzelhető, de mindent megteszünk, hogy Ön a lehető leghamarabb jusson hozzá a termékhez.
A termék adatai:
- Kiadás sorszáma 1st ed. 2020
- Kiadó Springer
- Megjelenés dátuma 2021. február 14.
- Kötetek száma 1 pieces, Book
- ISBN 9783030367237
- Kötéstípus Puhakötés
- Terjedelem760 oldal
- Méret 254x178 mm
- Súly 1480 g
- Nyelv angol
- Illusztrációk 172 Illustrations, black & white; 35 Illustrations, color 711
Kategóriák
Rövid leírás:
This book describes how neural networks operate from the mathematical point of view. As a result, neural networks can be interpreted both as function universal approximators and information processors. The book bridges the gap between ideas and concepts of neural networks, which are used nowadays at an intuitive level, and the precise modern mathematical language, presenting the best practices of the former and enjoying the robustness and elegance of the latter.
This book can be used in a graduate course in deep learning, with the first few parts being accessible to senior undergraduates. In addition, the book will be of wide interest to machine learning researchers who are interested in a theoretical understanding of the subject.
Több
Hosszú leírás:
This book describes how neural networks operate from the mathematical point of view. As a result, neural networks can be interpreted both as function universal approximators and information processors. The book bridges the gap between ideas and concepts of neural networks, which are used nowadays at an intuitive level, and the precise modern mathematical language, presenting the best practices of the former and enjoying the robustness and elegance of the latter.
This book can be used in a graduate course in deep learning, with the first few parts being accessible to senior undergraduates. In addition, the book will be of wide interest to machine learning researchers who are interested in a theoretical understanding of the subject.
?This book is useful to students who have already had an introductory course in machine learning and are further interested to deepen their understanding of the machine learning material from the mathematical point of view.? (T. C. Mohan, zbMATH 1441.68001, 2020)
TöbbTartalomjegyzék:
Introductory Problems.- Activation Functions.- Cost Functions.- Finding Minima Algorithms.- Abstract Neurons.- Neural Networks.- Approximation Theorems.- Learning with One-dimensional Inputs.- Universal Approximators.- Exact Learning.- Information Representation.- Information Capacity Assessment.- Output Manifolds.- Neuromanifolds.- Pooling.- Convolutional Networks.- Recurrent Neural Networks.- Classification.- Generative Models.- Stochastic Networks.- Hints and Solutions.
Több