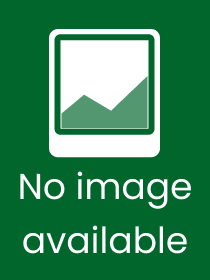
ISBN13: | 9783031413360 |
ISBN10: | 3031413369 |
Binding: | Hardback |
No. of pages: | 391 pages |
Size: | 235x155 mm |
Weight: | 876 g |
Language: | English |
Illustrations: | 45 Illustrations, black & white; 99 Illustrations, color |
654 |
Fundamentals of Supervised Machine Learning
EUR 128.39
Click here to subscribe.
Not in stock at Prospero.
This book presents the fundamental theoretical notions of supervised machine learning along with a wide range of applications using Python, R, and Stata. It provides a balance between theory and applications and fosters an understanding and awareness of the availability of machine learning methods over different software platforms.
After introducing the machine learning basics, the focus turns to a broad spectrum of topics: model selection and regularization, discriminant analysis, nearest neighbors, support vector machines, tree modeling, artificial neural networks, deep learning, and sentiment analysis. Each chapter is self-contained and comprises an initial theoretical part, where the basics of the methodologies are explained, followed by an applicative part, where the methods are applied to real-world datasets. Numerous examples are included and, for ease of reproducibility, the Python, R, and Stata codes used in the text, along with the related datasets, are available online.
The intended audience is PhD students, researchers and practitioners from various disciplines, including economics and other social sciences, medicine and epidemiology, who have a good understanding of basic statistics and a working knowledge of statistical software, and who want to apply machine learning methods in their work.
This book presents the fundamental theoretical notions of supervised machine learning along with a wide range of applications using Python, R, and Stata. It provides a balance between theory and applications and fosters an understanding and awareness of the availability of machine learning methods over different software platforms.
After introducing the machine learning basics, the focus turns to a broad spectrum of topics: model selection and regularization, discriminant analysis, nearest neighbors, support vector machines, tree modeling, artificial neural networks, deep learning, and sentiment analysis. Each chapter is self-contained and comprises an initial theoretical part, where the basics of the methodologies are explained, followed by an applicative part, where the methods are applied to real-world datasets. Numerous examples are included and, for ease of reproducibility, the Python, R, and Stata codes used in the text, along with the related datasets, are available online.
The intended audience is PhD students, researchers and practitioners from various disciplines, including economics and other social sciences, medicine and epidemiology, who have a good understanding of basic statistics and a working knowledge of statistical software, and who want to apply machine learning methods in their work.