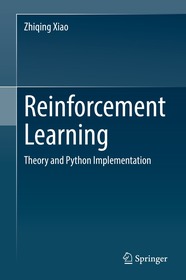
Reinforcement Learning
Theory and Python Implementation
-
20% KEDVEZMÉNY?
- A kedvezmény csak az 'Értesítés a kedvenc témákról' hírlevelünk címzettjeinek rendeléseire érvényes.
- Kiadói listaár EUR 80.24
-
Az ár azért becsült, mert a rendelés pillanatában nem lehet pontosan tudni, hogy a beérkezéskor milyen lesz a forint árfolyama az adott termék eredeti devizájához képest. Ha a forint romlana, kissé többet, ha javulna, kissé kevesebbet kell majd fizetnie.
- Kedvezmény(ek) 20% (cc. 6 807 Ft off)
- Discounted price 27 229 Ft (25 933 Ft + 5% áfa)
34 037 Ft
Beszerezhetőség
Becsült beszerzési idő: A Prosperónál jelenleg nincsen raktáron, de a kiadónál igen. Beszerzés kb. 3-5 hét..
A Prosperónál jelenleg nincsen raktáron.
Why don't you give exact delivery time?
A beszerzés időigényét az eddigi tapasztalatokra alapozva adjuk meg. Azért becsült, mert a terméket külföldről hozzuk be, így a kiadó kiszolgálásának pillanatnyi gyorsaságától is függ. A megadottnál gyorsabb és lassabb szállítás is elképzelhető, de mindent megteszünk, hogy Ön a lehető leghamarabb jusson hozzá a termékhez.
A termék adatai:
- Kiadás sorszáma 2024
- Kiadó Springer
- Megjelenés dátuma 2024. szeptember 29.
- Kötetek száma 1 pieces, Book
- ISBN 9789811949326
- Kötéstípus Keménykötés
- Terjedelem559 oldal
- Méret 235x155 mm
- Nyelv angol
- Illusztrációk 1 Illustrations, black & white; 60 Illustrations, color 641
Kategóriák
Rövid leírás:
Reinforcement Learning: Theory and Python Implementation is a tutorial book on reinforcement learning, with explanations of both theory and applications. Starting from a uniform mathematical framework, this book derives the theory of modern reinforcement learning systematically and introduces all mainstream reinforcement learning algorithms such as PPO, SAC, and MuZero. It also covers key technologies of GPT training such as RLHF, IRL, and PbRL. Every chapter is accompanied by high-quality implementations, and all implementations of deep reinforcement learning algorithms are with both TensorFlow and PyTorch. Codes can be found on GitHub along with their results and are runnable on a conventional laptop with either Windows, macOS, or Linux.
This book is intended for readers who want to learn reinforcement learning systematically and apply reinforcement learning to practical applications. It is also ideal to academical researchers who seek theoretical foundation or algorithm enhancement in their cutting-edge AI research.
TöbbHosszú leírás:
Reinforcement Learning: Theory and Python Implementation is a tutorial book on reinforcement learning, with explanations of both theory and applications. Starting from a uniform mathematical framework, this book derives the theory of modern reinforcement learning systematically and introduces all mainstream reinforcement learning algorithms such as PPO, SAC, and MuZero. It also covers key technologies of GPT training such as RLHF, IRL, and PbRL. Every chapter is accompanied by high-quality implementations, and all implementations of deep reinforcement learning algorithms are with both TensorFlow and PyTorch. Codes can be found on GitHub along with their results and are runnable on a conventional laptop with either Windows, macOS, or Linux.
This book is intended for readers who want to learn reinforcement learning systematically and apply reinforcement learning to practical applications. It is also ideal to academical researchers who seek theoretical foundation or algorithm enhancement in their cutting-edge AI research.
TöbbTartalomjegyzék:
Chapter 1. Introduction of Reinforcement Learning (RL).- Chapter 2. MDP: Markov Decision Process.- Chapter 3. Model-based Numerical Iteration.- Chapter 4. MC: Monte Carlo Learning.- Chapter 5. TD: Temporal Difference Learning.- Chapter 6. Function Approximation.- Chapter 7. PG: Policy Gradient.- Chapter 8. AC: Actor?Critic.- Chapter 9. DPG: Deterministic Policy Gradient.- Chapter 10. Maximum-Entropy RL.- Chapter 11. Policy-based Gradient-Free Algorithms.- Chapter 12. Distributional RL.- Chapter 13. Minimize Regret.- Chapter 14. Tree Search.- Chapter 15. More Agent?Environment Interfaces.- Chapter 16. Learn from Feedback and Imitation Learning.
Több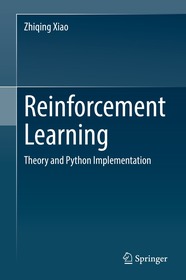
Reinforcement Learning: Theory and Python Implementation
Iratkozzon fel most és részesüljön kedvezőbb árainkból!
Feliratkozom
34 037 Ft
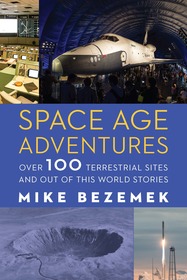
Space Age Adventures ? Over 100 Terrestrial Sites and Out of This World Stories: Over 100 Terrestrial Sites and Out of This World Stories
Iratkozzon fel most és részesüljön kedvezőbb árainkból!
Feliratkozom
11 129 Ft