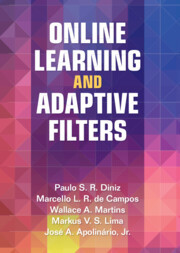
A termék adatai:
ISBN13: | 9781108842129 |
ISBN10: | 1108842127 |
Kötéstípus: | Keménykötés |
Terjedelem: | 300 oldal |
Méret: | 251x175x19 mm |
Súly: | 630 g |
Nyelv: | angol |
544 |
Témakör:
Online Learning and Adaptive Filters
Kiadó: Cambridge University Press
Megjelenés dátuma: 2022. december 8.
Normál ár:
Kiadói listaár:
GBP 79.99
GBP 79.99
Az Ön ára:
34 771 (33 116 Ft + 5% áfa )
Kedvezmény(ek): 10% (kb. 3 864 Ft)
A kedvezmény csak az 'Értesítés a kedvenc témákról' hírlevelünk címzettjeinek rendeléseire érvényes.
Kattintson ide a feliratkozáshoz
Kattintson ide a feliratkozáshoz
Beszerezhetőség:
Becsült beszerzési idő: A kiadót ért kibertámadás miatt a kiszolgálás várhatóan 2024. július végére áll helyre.2024.
A Prosperónál jelenleg nincsen raktáron.
Nem tudnak pontosabbat?
A Prosperónál jelenleg nincsen raktáron.
Rövid leírás:
Discover up-to-date techniques and algorithms in this concise, intuitive text, with extensive solutions for challenging learning problems.
Hosszú leírás:
Learn to solve the unprecedented challenges facing Online Learning and Adaptive Signal Processing in this concise, intuitive text. The ever-increasing amount of data generated every day requires new strategies to tackle issues such as: combining data from a large number of sensors; improving spectral usage, utilizing multiple-antennas with adaptive capabilities; or learning from signals placed on graphs, generating unstructured data. Solutions to all of these and more are described in a condensed and unified way, enabling you to expose valuable information from data and signals in a fast and economical way. The up-to-date techniques explained here can be implemented in simple electronic hardware, or as part of multi-purpose systems. Also featuring alternative explanations for online learning, including newly developed methods and data selection, and several easily implemented algorithms, this one-of-a-kind book is an ideal resource for graduate students, researchers, and professionals in online learning and adaptive filtering.
Tartalomjegyzék:
1. Introduction; 2. Adaptive filtering for sparse models; 3. Kernel-based adaptive filtering; 4. Distributed adaptive filters; 5. Adaptive beamforming; 6. Adaptive filtering on graphs.