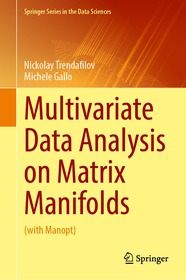
Multivariate Data Analysis on Matrix Manifolds
(with Manopt)
Sorozatcím: Springer Series in the Data Sciences;
-
8% KEDVEZMÉNY?
- A kedvezmény csak az 'Értesítés a kedvenc témákról' hírlevelünk címzettjeinek rendeléseire érvényes.
- Kiadói listaár EUR 53.49
-
Az ár azért becsült, mert a rendelés pillanatában nem lehet pontosan tudni, hogy a beérkezéskor milyen lesz a forint árfolyama az adott termék eredeti devizájához képest. Ha a forint romlana, kissé többet, ha javulna, kissé kevesebbet kell majd fizetnie.
- Kedvezmény(ek) 8% (cc. 1 815 Ft off)
- Discounted price 20 874 Ft (19 880 Ft + 5% áfa)
22 690 Ft
Beszerezhetőség
Becsült beszerzési idő: A Prosperónál jelenleg nincsen raktáron, de a kiadónál igen. Beszerzés kb. 3-5 hét..
A Prosperónál jelenleg nincsen raktáron.
Why don't you give exact delivery time?
A beszerzés időigényét az eddigi tapasztalatokra alapozva adjuk meg. Azért becsült, mert a terméket külföldről hozzuk be, így a kiadó kiszolgálásának pillanatnyi gyorsaságától is függ. A megadottnál gyorsabb és lassabb szállítás is elképzelhető, de mindent megteszünk, hogy Ön a lehető leghamarabb jusson hozzá a termékhez.
A termék adatai:
- Kiadás sorszáma 1st ed. 2021
- Kiadó Springer
- Megjelenés dátuma 2021. szeptember 16.
- Kötetek száma 1 pieces, Book
- ISBN 9783030769734
- Kötéstípus Keménykötés
- Terjedelem450 oldal
- Méret 235x155 mm
- Súly 955 g
- Nyelv angol
- Illusztrációk 1 Illustrations, black & white; 5 Illustrations, color 296
Kategóriák
Rövid leírás:
This graduate-level textbook aims to give a unified presentation and solution of several commonly used techniques for multivariate data analysis (MDA). Unlike similar texts, it treats the MDA problems as optimization problems on matrix manifolds defined by the MDA model parameters, allowing them to be solved using (free) optimization software Manopt. The book includes numerous in-text examples as well as Manopt codes and software guides, which can be applied directly or used as templates for solving similar and new problems. The first two chapters provide an overview and essential background for studying MDA, giving basic information and notations. Next, it considers several sets of matrices routinely used in MDA as parameter spaces, along with their basic topological properties. A brief introduction to matrix (Riemannian) manifolds and optimization methods on them with Manopt complete the MDA prerequisite. The remaining chapters study individual MDA techniques in depth. The number ofexercises complement the main text with additional information and occasionally involve open and/or challenging research questions. Suitable fields include computational statistics, data analysis, data mining and data science, as well as theoretical computer science, machine learning and optimization. It is assumed that the readers have some familiarity with MDA and some experience with matrix analysis, computing, and optimization.
TöbbHosszú leírás:
This graduate-level textbook aims to give a unified presentation and solution of several commonly used techniques for multivariate data analysis (MDA). Unlike similar texts, it treats the MDA problems as optimization problems on matrix manifolds defined by the MDA model parameters, allowing them to be solved using (free) optimization software Manopt. The book includes numerous in-text examples as well as Manopt codes and software guides, which can be applied directly or used as templates for solving similar and new problems. The first two chapters provide an overview and essential background for studying MDA, giving basic information and notations. Next, it considers several sets of matrices routinely used in MDA as parameter spaces, along with their basic topological properties. A brief introduction to matrix (Riemannian) manifolds and optimization methods on them with Manopt complete the MDA prerequisite. The remaining chapters study individual MDA techniques in depth. The number ofexercises complement the main text with additional information and occasionally involve open and/or challenging research questions. Suitable fields include computational statistics, data analysis, data mining and data science, as well as theoretical computer science, machine learning and optimization. It is assumed that the readers have some familiarity with MDA and some experience with matrix analysis, computing, and optimization.
TöbbTartalomjegyzék:
Introduction.- Matrix analysis and differentiation.- Matrix manifolds in MDA.- Principal component analysis (PCA).- Factor analysis (FA).- Procrustes analysis (PA).- Linear discriminant analysis (LDA).- Canonical correlation analysis (CCA).- Common principal components (CPC).- Metric multidimensional scaling (MDS) and related methods.- Data analysis on simplexes.
Több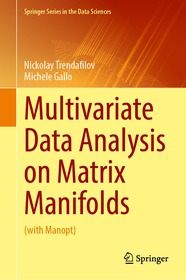
Multivariate Data Analysis on Matrix Manifolds: (with Manopt)
Iratkozzon fel most és részesüljön kedvezőbb árainkból!
Feliratkozom
22 690 Ft