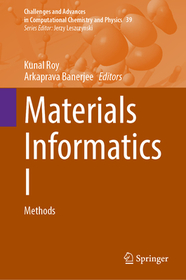
Materials Informatics I
Methods
Sorozatcím: Challenges and Advances in Computational Chemistry and Physics; 39;
-
8% KEDVEZMÉNY?
- A kedvezmény csak az 'Értesítés a kedvenc témákról' hírlevelünk címzettjeinek rendeléseire érvényes.
- Kiadói listaár EUR 267.49
-
Az ár azért becsült, mert a rendelés pillanatában nem lehet pontosan tudni, hogy a beérkezéskor milyen lesz a forint árfolyama az adott termék eredeti devizájához képest. Ha a forint romlana, kissé többet, ha javulna, kissé kevesebbet kell majd fizetnie.
- Kedvezmény(ek) 8% (cc. 9 078 Ft off)
- Discounted price 104 391 Ft (99 420 Ft + 5% áfa)
113 469 Ft
Beszerezhetőség
Még nem jelent meg, de rendelhető. A megjelenéstől számított néhány héten belül megérkezik.
Why don't you give exact delivery time?
A beszerzés időigényét az eddigi tapasztalatokra alapozva adjuk meg. Azért becsült, mert a terméket külföldről hozzuk be, így a kiadó kiszolgálásának pillanatnyi gyorsaságától is függ. A megadottnál gyorsabb és lassabb szállítás is elképzelhető, de mindent megteszünk, hogy Ön a lehető leghamarabb jusson hozzá a termékhez.
A termék adatai:
- Kiadó Springer
- Megjelenés dátuma 2025. április 3.
- Kötetek száma 1 pieces, Book
- ISBN 9783031787355
- Kötéstípus Keménykötés
- Terjedelem288 oldal
- Méret 235x155 mm
- Nyelv angol
- Illusztrációk 13 Illustrations, black & white; 53 Illustrations, color 700
Kategóriák
Rövid leírás:
This contributed volume explores the integration of machine learning and cheminformatics within materials science, focusing on predictive modeling techniques. It begins with foundational concepts in materials informatics and cheminformatics, emphasizing quantitative structure-property relationships (QSPR). The volume then presents various methods and tools, including advanced QSPR models, quantitative read-across structure-property relationship (q-RASPR) models, optimization strategies with minimal data, and in silico studies using different descriptors. Additionally, it explores machine learning algorithms and their applications in materials science, alongside innovative modeling approaches for quantum-theoretic properties. Overall, the book serves as a comprehensive resource for understanding and applying machine learning in the study and development of advanced materials and is a useful tool for students, researchers and professionals working in these areas.
TöbbHosszú leírás:
This contributed volume explores the integration of machine learning and cheminformatics within materials science, focusing on predictive modeling techniques. It begins with foundational concepts in materials informatics and cheminformatics, emphasizing quantitative structure-property relationships (QSPR). The volume then presents various methods and tools, including advanced QSPR models, quantitative read-across structure-property relationship (q-RASPR) models, optimization strategies with minimal data, and in silico studies using different descriptors. Additionally, it explores machine learning algorithms and their applications in materials science, alongside innovative modeling approaches for quantum-theoretic properties. Overall, the book serves as a comprehensive resource for understanding and applying machine learning in the study and development of advanced materials and is a useful tool for students, researchers and professionals working in these areas.
TöbbTartalomjegyzék:
Part 1. Introduction.- Introduction to Materials Informatics.- Introduction to Cheminformatics for Predictive Modeling.- Introduction to machine learning for predictive modeling of organic materials.- Quantitative Structure-Property Relationships (QSPR) for Materials Science.- Part 2. Methods and Tools.- Quantitative Structure-Property Relationships (QSPR) and Machine Learning (ML) Models for Materials Science.- Optimising Materials Properties with Minimal Data: Lessons from Vanadium Catalyst Modelling.- In silico QSPR studies based on CDFT and IT descriptors.- Applications of quantitative read-across structure-property relationship (q-RASPR) modeling in the field of materials science.- Machine Learning algorithms for applications in Materials Science I.- Machine Learning algorithms for applications in Materials Science II.- Structure-property modeling of quantum-theoretic properties of benzenoid hydrocarbons by means of connection-related graphical descriptors.- Machine learning tools and Web services for Materials Science modelling.
Több