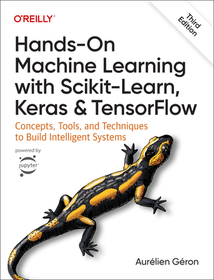
Hands?On Machine Learning with Scikit?Learn, Keras , and TensorFlow 3e
Concepts, Tools, and Techniques to Build Intelligent Systems
-
10% KEDVEZMÉNY?
- A kedvezmény csak az 'Értesítés a kedvenc témákról' hírlevelünk címzettjeinek rendeléseire érvényes.
- Kiadói listaár GBP 71.99
-
Az ár azért becsült, mert a rendelés pillanatában nem lehet pontosan tudni, hogy a beérkezéskor milyen lesz a forint árfolyama az adott termék eredeti devizájához képest. Ha a forint romlana, kissé többet, ha javulna, kissé kevesebbet kell majd fizetnie.
- Kedvezmény(ek) 10% (cc. 3 643 Ft off)
- Discounted price 32 791 Ft (31 229 Ft + 5% áfa)
36 434 Ft
Beszerezhetőség
Becsült beszerzési idő: A Prosperónál jelenleg nincsen raktáron, de a kiadónál igen. Beszerzés kb. 3-5 hét..
A Prosperónál jelenleg nincsen raktáron.
Why don't you give exact delivery time?
A beszerzés időigényét az eddigi tapasztalatokra alapozva adjuk meg. Azért becsült, mert a terméket külföldről hozzuk be, így a kiadó kiszolgálásának pillanatnyi gyorsaságától is függ. A megadottnál gyorsabb és lassabb szállítás is elképzelhető, de mindent megteszünk, hogy Ön a lehető leghamarabb jusson hozzá a termékhez.
A termék adatai:
- Kiadás sorszáma 3
- Kiadó O'Reilly
- Megjelenés dátuma 2022. október 18.
- Kötetek száma Print PDF
- ISBN 9781098125974
- Kötéstípus Puhakötés
- Terjedelem415 oldal
- Méret 232x194x46 mm
- Súly 1446 g
- Nyelv angol 1815
Kategóriák
Hosszú leírás:
Through a recent series of breakthroughs, deep learning has boosted the entire field of machine learning. Now, even programmers who know close to nothing about this technology can use simple, efficient tools to implement programs capable of learning from data. This best-selling book uses concrete examples, minimal theory, and production-ready Python frameworks--scikit-learn, Keras, and TensorFlow--to help you gain an intuitive understanding of the concepts and tools for building intelligent systems.
With this updated third edition, author Aurelien Geron explores a range of techniques, starting with simple linear regression and progressing to deep neural networks. Numerous code examples and exercises throughout the book help you apply what you've learned. Programming experience is all you need to get started.
- Use scikit-learn to track an example machine learning project end to end
- Explore several models, including support vector machines, decision trees, random forests, and ensemble methods
- Exploit unsupervised learning techniques such as dimensionality reduction, clustering, and anomaly detection
- Dive into neural net architectures, including convolutional nets, recurrent nets, generative adversarial networks, and transformers
- Use TensorFlow and Keras to build and train neural nets for computer vision, natural language processing, generative models, and deep reinforcement learning
- Train neural nets using multiple GPUs and deploy them at scale using Google's Vertex AI