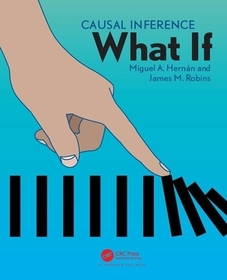
-
20% KEDVEZMÉNY?
- A kedvezmény csak az 'Értesítés a kedvenc témákról' hírlevelünk címzettjeinek rendeléseire érvényes.
- Kiadói listaár GBP 41.99
-
Az ár azért becsült, mert a rendelés pillanatában nem lehet pontosan tudni, hogy a beérkezéskor milyen lesz a forint árfolyama az adott termék eredeti devizájához képest. Ha a forint romlana, kissé többet, ha javulna, kissé kevesebbet kell majd fizetnie.
- Kedvezmény(ek) 20% (cc. 4 250 Ft off)
- Discounted price 17 001 Ft (16 191 Ft + 5% áfa)
21 251 Ft
Beszerezhetőség
Még nem jelent meg, de rendelhető. A megjelenéstől számított néhány héten belül megérkezik.
Why don't you give exact delivery time?
A beszerzés időigényét az eddigi tapasztalatokra alapozva adjuk meg. Azért becsült, mert a terméket külföldről hozzuk be, így a kiadó kiszolgálásának pillanatnyi gyorsaságától is függ. A megadottnál gyorsabb és lassabb szállítás is elképzelhető, de mindent megteszünk, hogy Ön a lehető leghamarabb jusson hozzá a termékhez.
A termék adatai:
- Kiadás sorszáma 1
- Kiadó CRC Press
- Megjelenés dátuma 2025. július 30.
- ISBN 9781420076165
- Kötéstípus Keménykötés
- Terjedelem312 oldal
- Méret 280x210 mm
- Súly 453 g
- Nyelv angol
- Illusztrációk 128 Illustrations, black & white; 128 Line drawings, black & white; 21 Tables, black & white 700
Kategóriák
Rövid leírás:
Written by pioneers in the field, this practical book presents an authoritative yet accessible overview of the methods and applications of causal inference. The text provides a thorough introduction to the basics of the theory for non-time-varying treatments and the generalization to complex longitudinal data.
TöbbHosszú leírás:
Causal inference is a complex scientific task that relies on evidence from multiple sources and a variety of
methodological approaches. By providing a cohesive presentation of concepts and methods that are currently
scattered across journals in several disciplines, Causal Inference: What If provides an introduction to causal
inference for scientists who design studies and analyze data. The book is divided into three parts of increasing
difficulty: causal inference without models, causal inference with models, and causal inference from complex
longitudinal data.
FEATURES:
? Emphasizes taking the causal question seriously enough to articulate it with sufficient precision
? Shows that causal inference from observational data relies on subject-matter knowledge and therefore
cannot be reduced to a collection of recipes for data analysis
? Describes causal diagrams, both directed acyclic graphs and single-world intervention graphs
? Explains various data analysis approaches to estimate causal effects from individual-level data, including
the g-formula, inverse probability weighting, g-estimation, instrumental variable estimation, outcome
regression, and propensity score adjustment
? Includes software and real data examples, as well as ?Fine Points? and ?Technical Points? throughout to
elaborate on certain key topics
Causal Inference: What If has been written for all scientists that make causal inferences, including epidemiologists,
statisticians, psychologists, economists, sociologists, political scientists, computer scientists, and more. The book is substantially class-tested,
as it has been used in dozens of universities to teach courses on causal inference at graduate and advanced undergraduate level.
"With this clear rigorous, and readable presentation of models for causal inference using potential
outcomes and counterfactuals, Hernan and Robins have provided a text that will be useful and enjoyable
for students, practitioners, and researchers in statistics and applied fields."
- Andrew Gelman, Columbia University, USA
?This is the definitive book on modeling causal effects and conducting statistical inference with
the resulting models, invaluable both as teaching and reference resource. It brings together a vast
range of developments over recent decades in a well-organized manner, with exceptionally clear descriptions
of the models, methods, emphasizing their motivations in scientific questions and goals. Especially valuable
are the careful links drawn from everyday notions of causal and selection effects to modern causal models,
including models for estimating and optimizing effects of chronic treatments ? those administered over
sustained periods of time, as are now standard in modern preventive medicine.?
? Sander Greenland, Emeritus Professor of Epidemiology and Statistics, University of
California Los Angeles
"This is the most eagerly anticipated book ever in its field. It provides a lean, readable, comprehensive
and coherent formulation of methods for strengthening causal inference in (largely) non-experimental data.
In crystal clear language it takes the reader through the logic and practice of setting up and analysing a
causal question, and, if taken literally, should reveal the many situations when the data available to the
investigator do not provide an adequate basis for asking specific causal questions. It provides the ideal core
text for a course on causal inference that will be of relevance to many disciplines. The book has been carefully
hewn over a decade through public scrutiny, response and revision, with drafts having been made available on-line
and, in addition to the fabulous content, it will surely be seen as a truly innovative model for future book
production. Because of its unique pre-publication history it has become a citation classic over the last 10 years.
I can think of few epidemiologists who will not want to own their own copy of this book, and this will likely
apply to investigators in many other fields."
? George Davey Smith FRS, Director of the Medical Research Council Integrative Epidemiology
Unit (IEU), University of Bristol, UK
"Hernán and Robins have contributed enormously to the concepts and methods of causal inference. Now their
unique collaboration has culminated in an invaluable gift to any researcher in the biomedical or social sciences,
a Rosetta Stone for causal inference. The ideas are gathered from myriad sources in diverse disciplines, and
woven into one coherent package of logical exposition. In part I, Causal Inference Without Models, they elucidate
how to frame a causal question so that it is answerable, while spotlighting the assumptions needed to reach a
correct inference. In part II, they progress to using parametric modeling, and in part III they explore the
intricacies of evaluating causal questions using complex longitudinal data. The language, reflecting the thinking,
is direct and clear. With this work, they have performed a great service for generations of students and researchers."
- Kenneth Rothman, Boston University, USA
"Anyone interested in data science and machine learning should read this book. To form valid models of the world
from data, understanding causal inference is critical. They?ve provided a comprehensive and accessible introduction
to the topic."
- Suchi Saria, John Hopkins University, USA
Tartalomjegyzék:
Part I: Causal inference without models 1. A definition of causal effect 2. Randomized experiments 3. Observational studies 4. Effect modification
5. Interaction 6. Graphical representation of causal effects 7. Confounding 8. Selection bias
9. Measurement bias 10. Random variability Part II: Causal inference with models 11. Why model?
12. IP weighting and marginal structural models 13. Standardization and the parametric g-formula
14. G-estimation of structural nested models 15 Outcome regression and propensity scores
16. Instrumental variable estimation 17. Causal survival analysis 18 Variable selection for causal inference
Part III: Causal inference from complex longitudinal data 19. Time-varying treatments
20. Treatment-confounder feedback 21. G-methods for time-varying treatments 22. Target trial emulation
23. Causal mediation
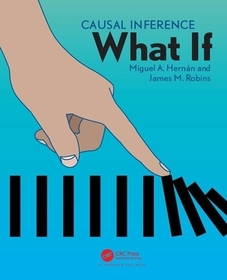
Causal Inference: What If
Iratkozzon fel most és részesüljön kedvezőbb árainkból!
Feliratkozom
21 251 Ft
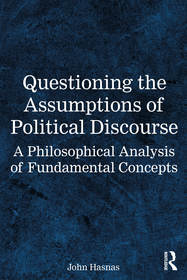
Questioning the Assumptions of Political Discourse: A Philosophical Analysis of Fundamental Concepts
Iratkozzon fel most és részesüljön kedvezőbb árainkból!
Feliratkozom
20 238 Ft