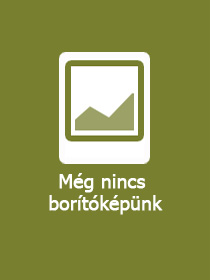
A termék adatai:
ISBN13: | 9783031573880 |
ISBN10: | 3031573889 |
Kötéstípus: | Keménykötés |
Terjedelem: | 153 oldal |
Méret: | 235x155 mm |
Nyelv: | angol |
Illusztrációk: | 2 Illustrations, black & white; 56 Illustrations, color |
700 |
Témakör:
Backdoor Attacks against Learning-Based Algorithms
Sorozatcím:
Wireless Networks;
Kiadás sorszáma: 1st ed. 2024
Kiadó: Springer
Megjelenés dátuma: 2024. június 15.
Kötetek száma: 1 pieces, Book
Normál ár:
Kiadói listaár:
EUR 160.49
EUR 160.49
Az Ön ára:
52 980 (50 458 Ft + 5% áfa )
Kedvezmény(ek): 20% (kb. 13 245 Ft)
A kedvezmény érvényes eddig: 2024. június 30.
A kedvezmény csak az 'Értesítés a kedvenc témákról' hírlevelünk címzettjeinek rendeléseire érvényes.
Kattintson ide a feliratkozáshoz
Kattintson ide a feliratkozáshoz
Beszerezhetőség:
Még nem jelent meg, de rendelhető. A megjelenéstől számított néhány héten belül megérkezik.
Rövid leírás:
This book introduces a new type of data poisoning attack, dubbed, backdoor attack. In backdoor attacks, an attacker can train the model with poisoned data to obtain a model that performs well on a normal input but behaves wrongly with crafted triggers. Backdoor attacks can occur in many scenarios where the training process is not entirely controlled, such as using third-party datasets, third-party platforms for training, or directly calling models provided by third parties. Due to the enormous threat that backdoor attacks pose to model supply chain security, they have received widespread attention from academia and industry. This book focuses on exploiting backdoor attacks in the three types of DNN applications, which are image classification, natural language processing, and federated learning.
This book introduces a new type of data poisoning attack, dubbed, backdoor attack. In backdoor attacks, an attacker can train the model with poisoned data to obtain a model that performs well on a normal input but behaves wrongly with crafted triggers. Backdoor attacks can occur in many scenarios where the training process is not entirely controlled, such as using third-party datasets, third-party platforms for training, or directly calling models provided by third parties. Due to the enormous threat that backdoor attacks pose to model supply chain security, they have received widespread attention from academia and industry. This book focuses on exploiting backdoor attacks in the three types of DNN applications, which are image classification, natural language processing, and federated learning.
Based on the observation that DNN models are vulnerable to small perturbations, this book demonstrates that steganography and regularization can be adopted to enhance the invisibility of backdoor triggers. Based on image similarity measurement, this book presents two metrics to quantitatively measure the invisibility of backdoor triggers. The invisible trigger design scheme introduced in this book achieves a balance between the invisibility and the effectiveness of backdoor attacks. In the natural language processing domain, it is difficult to design and insert a general backdoor in a manner imperceptible to humans. Any corruption to the textual data (e.g., misspelled words or randomly inserted trigger words/sentences) must retain context-awareness and readability to human inspectors. This book introduces two novel hidden backdoor attacks, targeting three major natural language processing tasks, including toxic comment detection, neural machine translation, and question answering, depending on whether the targeted NLP platform accepts raw Unicode characters.
The emerged distributed training framework, i.e., federated learning, has advantages in preserving users' privacy. It has been widely used in electronic medical applications, however, it also faced threats derived from backdoor attacks. This book presents a novel backdoor detection framework in FL-based e-Health systems. We hope this book can provide insightful lights on understanding the backdoor attacks in different types of learning-based algorithms, including computer vision, natural language processing, and federated learning. The systematic principle in this book also offers valuable guidance on the defense of backdoor attacks against future learning-based algorithms.
Hosszú leírás:
This book introduces a new type of data poisoning attack, dubbed, backdoor attack. In backdoor attacks, an attacker can train the model with poisoned data to obtain a model that performs well on a normal input but behaves wrongly with crafted triggers. Backdoor attacks can occur in many scenarios where the training process is not entirely controlled, such as using third-party datasets, third-party platforms for training, or directly calling models provided by third parties. Due to the enormous threat that backdoor attacks pose to model supply chain security, they have received widespread attention from academia and industry. This book focuses on exploiting backdoor attacks in the three types of DNN applications, which are image classification, natural language processing, and federated learning.
Based on the observation that DNN models are vulnerable to small perturbations, this book demonstrates that steganography and regularization can be adopted to enhance the invisibility of backdoor triggers. Based on image similarity measurement, this book presents two metrics to quantitatively measure the invisibility of backdoor triggers. The invisible trigger design scheme introduced in this book achieves a balance between the invisibility and the effectiveness of backdoor attacks. In the natural language processing domain, it is difficult to design and insert a general backdoor in a manner imperceptible to humans. Any corruption to the textual data (e.g., misspelled words or randomly inserted trigger words/sentences) must retain context-awareness and readability to human inspectors. This book introduces two novel hidden backdoor attacks, targeting three major natural language processing tasks, including toxic comment detection, neural machine translation, and question answering, depending on whether the targeted NLP platform accepts raw Unicode characters.
The emerged distributed training framework, i.e., federated learning, has advantages in preserving users' privacy. It has been widely used in electronic medical applications, however, it also faced threats derived from backdoor attacks. This book presents a novel backdoor detection framework in FL-based e-Health systems. We hope this book can provide insightful lights on understanding the backdoor attacks in different types of learning-based algorithms, including computer vision, natural language processing, and federated learning. The systematic principle in this book also offers valuable guidance on the defense of backdoor attacks against future learning-based algorithms.
Tartalomjegyzék:
Introduction.- Literature Review of Backdoor Attacks.- Invisible Backdoor Attacks in Image Classification Based Network Services.- Hidden Backdoor Attacks in NLP Based Network Services.- Backdoor Attacks and Defense in FL.- Summary and Future Directions.