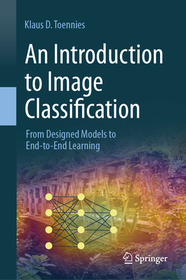
An Introduction to Image Classification
From Designed Models to End-to-End Learning
-
20% KEDVEZMÉNY?
- A kedvezmény csak az 'Értesítés a kedvenc témákról' hírlevelünk címzettjeinek rendeléseire érvényes.
- Kiadói listaár EUR 74.89
-
Az ár azért becsült, mert a rendelés pillanatában nem lehet pontosan tudni, hogy a beérkezéskor milyen lesz a forint árfolyama az adott termék eredeti devizájához képest. Ha a forint romlana, kissé többet, ha javulna, kissé kevesebbet kell majd fizetnie.
- Kedvezmény(ek) 20% (cc. 6 354 Ft off)
- Discounted price 25 414 Ft (24 204 Ft + 5% áfa)
31 768 Ft
Beszerezhetőség
Becsült beszerzési idő: A Prosperónál jelenleg nincsen raktáron, de a kiadónál igen. Beszerzés kb. 3-5 hét..
A Prosperónál jelenleg nincsen raktáron.
Why don't you give exact delivery time?
A beszerzés időigényét az eddigi tapasztalatokra alapozva adjuk meg. Azért becsült, mert a terméket külföldről hozzuk be, így a kiadó kiszolgálásának pillanatnyi gyorsaságától is függ. A megadottnál gyorsabb és lassabb szállítás is elképzelhető, de mindent megteszünk, hogy Ön a lehető leghamarabb jusson hozzá a termékhez.
A termék adatai:
- Kiadás sorszáma 1st ed. 2024
- Kiadó Springer
- Megjelenés dátuma 2024. január 25.
- Kötetek száma 1 pieces, Book
- ISBN 9789819978816
- Kötéstípus Keménykötés
- Terjedelem290 oldal
- Méret 235x155 mm
- Súly 629 g
- Nyelv angol
- Illusztrációk 1 Illustrations, black & white 573
Kategóriák
Rövid leírás:
Image classification is a critical component in computer vision tasks and has numerous applications. Traditional methods for image classification involve feature extraction and classification in feature space. Current state-of-the-art methods utilize end-to-end learning with deep neural networks, where feature extraction and classification are integrated into the model. Understanding traditional image classification is important because many of its design concepts directly correspond to components of a neural network. This knowledge can help demystify the behavior of these networks, which may seem opaque at first sight.
The book starts from introducing methods for model-driven feature extraction and classification, including basic computer vision techniques for extracting high-level semantics from images. A brief overview of probabilistic classification with generative and discriminative classifiers is then provided. Next, neural networks are presented as a means to learn a classification model directly from labeled sample images, with individual components of the network discussed. The relationships between network components and those of a traditional designed model are explored, and different concepts for regularizing model training are explained. Finally, various methods for analyzing what a network has learned are covered in the closing section of the book.
The topic of image classification is presented as a thoroughly curated sequence of steps that gradually increase understanding of the working of a fully trainable classifier. Practical exercises in Python/Keras/Tensorflow have been designed to allow for experimental exploration of these concepts. In each chapter, suitable functions from Python modules are briefly introduced to provide students with the necessary tools to conduct these experiments.
Több
Hosszú leírás:
Image classification is a critical component in computer vision tasks and has numerous applications. Traditional methods for image classification involve feature extraction and classification in feature space. Current state-of-the-art methods utilize end-to-end learning with deep neural networks, where feature extraction and classification are integrated into the model. Understanding traditional image classification is important because many of its design concepts directly correspond to components of a neural network. This knowledge can help demystify the behavior of these networks, which may seem opaque at first sight.
The book starts from introducing methods for model-driven feature extraction and classification, including basic computer vision techniques for extracting high-level semantics from images. A brief overview of probabilistic classification with generative and discriminative classifiers is then provided. Next, neural networks are presented as a means to learn a classification model directly from labeled sample images, with individual components of the network discussed. The relationships between network components and those of a traditional designed model are explored, and different concepts for regularizing model training are explained. Finally, various methods for analyzing what a network has learned are covered in the closing section of the book.
The topic of image classification is presented as a thoroughly curated sequence of steps that gradually increase understanding of the working of a fully trainable classifier. Practical exercises in Python/Keras/Tensorflow have been designed to allow for experimental exploration of these concepts. In each chapter, suitable functions from Python modules are briefly introduced to provide students with the necessary tools to conduct these experiments.
Több
Tartalomjegyzék:
Chapter 1. Image Classification ? A Computer Vision Task.- Chapter 2. Image Features ? Extraction and Categories.- Chapter 3. Feature Reduction.- Chapter 4. Bayesian Image Classification in Feature Space.- Chapter 5. Distance-based Classifiers.- Chapter 6. Decision Boundaries in Feature Space.- Chapter 7. Multi-layer Perceptron for Image Classification.- Chapter 8. Feature Extraction by Convolutional Neural Network.- Chapter 9. Network Set-up for Image Classification.- Chapter 10. Basic Network Training for Image Classification.- Chapter 11. Dealing with Training Deficiencies.- Chapter 12. Learning Effects and Network Decisions.
Több
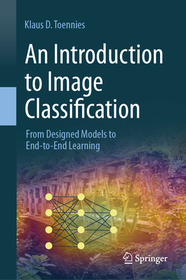
An Introduction to Image Classification: From Designed Models to End-to-End Learning
Iratkozzon fel most és részesüljön kedvezőbb árainkból!
Feliratkozom
31 768 Ft
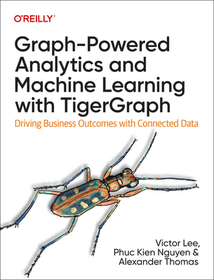
Graph?Powered Analytics and Machine Learning with TigerGraph
Iratkozzon fel most és részesüljön kedvezőbb árainkból!
Feliratkozom
26 818 Ft
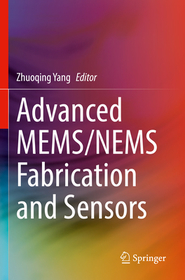
Advanced MEMS/NEMS Fabrication and Sensors
Iratkozzon fel most és részesüljön kedvezőbb árainkból!
Feliratkozom
63 540 Ft