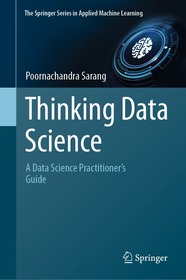
Thinking Data Science
EUR 69.54
Click here to subscribe.
Not in stock at Prospero.
ISBN13: | 9783031023620 |
ISBN10: | 3031023625 |
Binding: | Hardback |
No. of pages: | 358 pages |
Size: | 235x155 mm |
Weight: | 735 g |
Language: | English |
Illustrations: | 101 Illustrations, black & white; 132 Illustrations, color |
592 |
Probability and mathematical statistics
System analysis, system planning
Operating systems and graphical user interfaces
Artificial Intelligence
Probability and mathematical statistics (charity campaign)
System analysis, system planning (charity campaign)
Operating systems and graphical user interfaces (charity campaign)
Artificial Intelligence (charity campaign)
This definitive guide to Machine Learning projects answers the problems an aspiring or experienced data scientist frequently has: Confused on what technology to use for your ML development? Should I use GOFAI, ANN/DNN or Transfer Learning? Can I rely on AutoML for model development? What if the client provides me Gig and Terabytes of data for developing analytic models? How do I handle high-frequency dynamic datasets? This book provides the practitioner with a consolidation of the entire data science process in a single ?Cheat Sheet?.
The challenge for a data scientist is to extract meaningful information from huge datasets that will help to create better strategies for businesses. Many Machine Learning algorithms and Neural Networks are designed to do analytics on such datasets. For a data scientist, it is a daunting decision as to which algorithm to use for a given dataset. Although there is no single answer to this question, a systematic approach to problem solving is necessary. This book describes the various ML algorithms conceptually and defines/discusses a process in the selection of ML/DL models. The consolidation of available algorithms and techniques for designing efficient ML models is the key aspect of this book. Thinking Data Science will help practising data scientists, academicians, researchers, and students who want to build ML models using the appropriate algorithms and architectures, whether the data be small or big.This definitive guide to Machine Learning projects answers the problems an aspiring or experienced data scientist frequently has: Confused on what technology to use for your ML development? Should I use GOFAI, ANN/DNN or Transfer Learning? Can I rely on AutoML for model development? What if the client provides me Gig and Terabytes of data for developing analytic models? How do I handle high-frequency dynamic datasets? This book provides the practitioner with a consolidation of the entire data science process in a single ?Cheat Sheet?.
The challenge for a data scientist is to extract meaningful information from huge datasets that will help to create better strategies for businesses. Many Machine Learning algorithms and Neural Networks are designed to do analytics on such datasets. For a data scientist, it is a daunting decision as to which algorithm to use for a given dataset. Although there is no single answer to this question, a systematic approach to problem solving is necessary. This book describes the various ML algorithms conceptually and defines/discusses a process in the selection of ML/DL models. The consolidation of available algorithms and techniques for designing efficient ML models is the key aspect of this book. Thinking Data Science will help practising data scientists, academicians, researchers, and students who want to build ML models using the appropriate algorithms and architectures, whether the data be small or big.