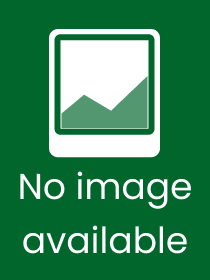
ISBN13: | 9781498796330 |
ISBN10: | 1498796338 |
Binding: | Hardback |
No. of pages: | 400 pages |
Size: | 234x156 mm |
Language: | English |
Illustrations: | 91 Illustrations, color; 91 Line drawings, color; 4 Tables, black & white |
700 |
Mathematics in general
Number theory
Analysis
Biology in general
Theory of computing, computing in general
Computer architecture, logic design
Supercomputers
Operating systems and graphical user interfaces
Physics in general
Mathematics in general (charity campaign)
Number theory (charity campaign)
Analysis (charity campaign)
Biology in general (charity campaign)
Theory of computing, computing in general (charity campaign)
Computer architecture, logic design (charity campaign)
Supercomputers (charity campaign)
Operating systems and graphical user interfaces (charity campaign)
Physics in general (charity campaign)
Stochastic Methods in Scientific Computing
GBP 89.99
Click here to subscribe.
This book introduces the reader to advanced concepts in stochastic modelling, rooted in an intuitive yet rigorous presentation of the underlying mathematical concepts. The reader will find valuable insights into topics ranging from Social Sciences and Particle Physics to modern-day Computer Science with Machine Learning and AI in focus.
Stochastic Methods in Scientific Computing: From Foundations to Advanced Techniques introduces the reader to advanced concepts in stochastic modelling, rooted in an intuitive yet rigorous presentation of the underlying mathematical concepts. A particular emphasis is placed on illuminating the underpinning Mathematics, and yet have the practical applications in mind. The reader will find valuable insights into topics ranging from Social Sciences and Particle Physics to modern-day Computer Science with Machine Learning and AI in focus. The book also covers recent specialised techniques for notorious issues in the field of stochastic simulations, providing a valuable reference for advanced readers with an active interest in the field.
Features
- Self-contained, starting from the theoretical foundations and advancing to the most recent developments in the field
- Suitable as a reference for post-graduates and researchers or as supplementary reading for courses in numerical methods, scientific computing, and beyond
- Interdisciplinary, laying a solid ground for field-specific applications in finance, physics and biosciences on common theoretical foundations
- Replete with practical examples of applications to classic and current research problems in various fields.
1. Random Numbers. 1.1. Random numbers and probability distribution. 1.2. Central limit theorem. 1.3. Beyond the Normal distribution. 1.4. Exercises. 2. Random walks. 2.1. Random walk as a Markov process. 2.2. Random walks in 1 and 2 dimensions. 2.3. Levy flight. 2.4. Random walks with potentials. 2.5. Exercises. 3. Monte Carlo methods. 3.1. Objectives and concepts. 3.2. Monte-Carlo integration. 3.3. Markov Chain Monte-Carlo. 3.4. Advanced Error Analysis Techniques. 3.5. Error estimate in the presence of autocorrelation. 3.6. Error estimate for non-trivial estimators: The Jackknife, and the Bootstrap. 3.7. Biased Estimators. 3.8. Exercises. 4. Statistical models. 4.1. An introduction to thermodynamics. 4.2. From thermodynamics to statistical mechanics. 4.3. Phase transitions. 4.4. The Ising model. 4.5. An overview of other models. 4.6. Exercises. 5. Advanced Monte-Carlo simulation techniques. 5.1. Hamiltonian (Hybrid) Monte-Carlo (HMC) simulations. 5.2. Non-local Monte-Carlo update. 5.3. Micro-canonical simulations. 5.4. Flat histogram methods. 5.5. The Linear Logarithmic Relaxation (LLR) method. 5.6. Exercises. 6. From Statistical Systems to Quantum Field Theory. 6.1. Invitation: The O(2) model. 6.2. The Bridge to QFT: the Feynman path-integral. 6.3. Gauge Theories. 6.4. Adding fermion fields. 6.5. Exercises. 7. Current challenges in Monte-Carlo Simulations. 7.1. Sign and overlap problems. 7.2. Introduction to overlap problems. 7.3. Estimating probability density functions. 8. Data Analytics and Statistical Systems. 8.1. Model regression - L2 norm. 8.2. Gaussian Process. 8.3. Machine learning with graphs. 8.4. Emulation of statistical systems with Machine Learning. 8.5. Categorisation in statistical physics: Naive Bayes. 8.6. Machine learning classification of phase transitions.