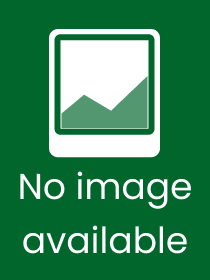
Machine Learning Safety
Series: Artificial Intelligence: Foundations, Theory, and Algorithms;
- Publisher's listprice EUR 74.89
-
The price is estimated because at the time of ordering we do not know what conversion rates will apply to HUF / product currency when the book arrives. In case HUF is weaker, the price increases slightly, in case HUF is stronger, the price goes lower slightly.
- Discount 8% (cc. 2 541 Ft off)
- Discounted price 29 226 Ft (27 835 Ft + 5% VAT)
Subcribe now and take benefit of a favourable price.
Subscribe
31 768 Ft
Availability
Estimated delivery time: In stock at the publisher, but not at Prospero's office. Delivery time approx. 3-5 weeks.
Not in stock at Prospero.
Why don't you give exact delivery time?
Delivery time is estimated on our previous experiences. We give estimations only, because we order from outside Hungary, and the delivery time mainly depends on how quickly the publisher supplies the book. Faster or slower deliveries both happen, but we do our best to supply as quickly as possible.
Product details:
- Edition number 2023
- Publisher Springer
- Date of Publication 29 April 2023
- Number of Volumes 1 pieces, Book
- ISBN 9789811968136
- Binding Hardback
- No. of pages321 pages
- Size 235x155 mm
- Weight 676 g
- Language English
- Illustrations 1 Illustrations, black & white 483
Categories
Short description:
Machine learning algorithms allow computers to learn without being explicitly programmed. Their application is now spreading to highly sophisticated tasks across multiple domains, such as medical diagnostics or fully autonomous vehicles. While this development holds great potential, it also raises new safety concerns, as machine learning has many specificities that make its behaviour prediction and assessment very different from that for explicitly programmed software systems. This book addresses the main safety concerns with regard to machine learning, including its susceptibility to environmental noise and adversarial attacks. Such vulnerabilities have become a major roadblock to the deployment of machine learning in safety-critical applications. The book presents up-to-date techniques for adversarial attacks, which are used to assess the vulnerabilities of machine learning models; formal verification, which is used to determine if a trained machine learning model is free of vulnerabilities; and adversarial training, which is used to enhance the training process and reduce vulnerabilities.
The book aims to improve readers? awareness of the potential safety issues regarding machine learning models. In addition, it includes up-to-date techniques for dealing with these issues, equipping readers with not only technical knowledge but also hands-on practical skills.
MoreLong description:
Machine learning algorithms allow computers to learn without being explicitly programmed. Their application is now spreading to highly sophisticated tasks across multiple domains, such as medical diagnostics or fully autonomous vehicles. While this development holds great potential, it also raises new safety concerns, as machine learning has many specificities that make its behaviour prediction and assessment very different from that for explicitly programmed software systems. This book addresses the main safety concerns with regard to machine learning, including its susceptibility to environmental noise and adversarial attacks. Such vulnerabilities have become a major roadblock to the deployment of machine learning in safety-critical applications. The book presents up-to-date techniques for adversarial attacks, which are used to assess the vulnerabilities of machine learning models; formal verification, which is used to determine if a trained machine learning model is free of vulnerabilities; and adversarial training, which is used to enhance the training process and reduce vulnerabilities.
The book aims to improve readers? awareness of the potential safety issues regarding machine learning models. In addition, it includes up-to-date techniques for dealing with these issues, equipping readers with not only technical knowledge but also hands-on practical skills.
?The authors are top researchers in the field. ... this book leaves a reader longing for more profound insights.? (Subhankar Ray, Computing Reviews, March 29, 2024)
MoreTable of Contents:
1. Introduction.- 2. Safety of Simple Machine Learning Models.- 3. Safety of Deep Learning.- 4. Robustness Verification of Deep Learning.- 5. Enhancement to Robustness and Generalization.- 6. Probabilistic Graph Model.- A. Mathematical Foundations.- B. Competitions.
More