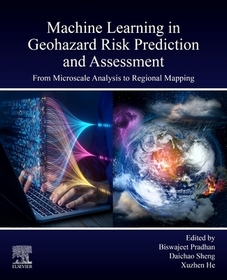
Machine Learning in Geohazard Risk Prediction and Assessment
From Microscale Analysis to Regional Mapping
- Publisher's listprice EUR 163.99
-
The price is estimated because at the time of ordering we do not know what conversion rates will apply to HUF / product currency when the book arrives. In case HUF is weaker, the price increases slightly, in case HUF is stronger, the price goes lower slightly.
- Discount 20% (cc. 13 913 Ft off)
- Discounted price 55 651 Ft (53 001 Ft + 5% VAT)
Subcribe now and take benefit of a favourable price.
Subscribe
69 564 Ft
Availability
Not yet published.
Why don't you give exact delivery time?
Delivery time is estimated on our previous experiences. We give estimations only, because we order from outside Hungary, and the delivery time mainly depends on how quickly the publisher supplies the book. Faster or slower deliveries both happen, but we do our best to supply as quickly as possible.
Product details:
- Publisher Elsevier
- Date of Publication 1 July 2025
- ISBN 9780443236631
- Binding Paperback
- No. of pages376 pages
- Size 235x191 mm
- Language English 700
Categories
Long description:
Machine Learning in Geohazard Risk Prediction and Assessment: From Microscale Analysis to Regional Mapping presents an overview of the most recent developments in machine learning techniques that have reshaped our understanding of geo-materials and management protocols of geo-risk. The book covers a broad category of research on machine-learning techniques that can be applied, from microscopic modeling to constitutive modeling, to physics-based numerical modeling, to regional susceptibility mapping. This is a good reference for researchers, academicians, graduate and undergraduate students, professionals, and practitioners in the field of geotechnical engineering and applied geology.
- Introduces machine-learning techniques in the risk management of geo-hazards, particularly recent developments
- Covers a broader category of research and machine-learning techniques that can be applied, from microscopic modeling to constitutive modeling, to physics-based numerical modeling, to regional susceptibility mapping
- Contains contributions from top researchers around the world, including authors from the UK, USA, Australia, Austria, China, and India
Table of Contents:
Part 1: Machine learning methods and connections between different parts.
1. Machine learning methods
2. Connections between studies across different scales
3. Summary and outlook
Part 2: Machine learning in microscopic modelling of geo-materials.
4. Machine-learning-enabled discrete element method
5. Machine learning in micromechanics based virtual laboratory testing
6. Integrating X-ray CT and machine learning for better understanding of granular materials
7. Summary and outlook
Part 3: Machine learning in constitutive modelling of geo-materials.
8. Thermodynamics-driven deep neural network as constitutive equations
9. Deep active learning for constitutive modelling of granular materials
10. Summary and outlook
Part 4: Machine learning in design of geo-structures.
11. Deep learning for surrogate modelling for geotechnical risk analysis
12. Deep learning for geotechnical optimization of designs
13. Deep learning for time series forecasting in geotechnical engineering
14. Summary and outlook
Part 5: Machine learning in geo-risk susceptibility mapping for regions of various sizes.
15. Deep learning and ensemble modeling of debris flows, mud flows and rockfalls.
16. Integrating machine learning and physical-based models in landslide susceptibility and hazard mapping.
17. Explainable AI (XAI) in landslide susceptibility, hazard, vulnerability and risk assessment.
18. New approaches for data collection for susceptibility mapping
19. Summary and outlook