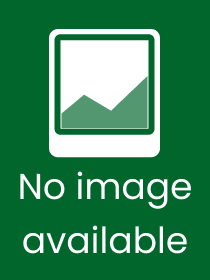
ISBN13: | 9789819920952 |
ISBN10: | 9819920957 |
Binding: | Hardback |
No. of pages: | 244 pages |
Size: | 235x155 mm |
Language: | English |
Illustrations: | 30 Illustrations, black & white; 53 Illustrations, color |
700 |
Machine Learning Assisted Evolutionary Multi- and Many- Objective Optimization
EUR 171.19
Click here to subscribe.
This book focuses on machine learning (ML) assisted evolutionary multi- and many-objective optimization (EMâO). EMâO algorithms, namely EMâOAs, iteratively evolve a set of solutions towards a good Pareto Front approximation. The availability of multiple solution sets over successive generations makes EMâOAs amenable to application of ML for different pursuits.
Recognizing the immense potential for ML-based enhancements in the EMâO domain, this book intends to serve as an exclusive resource for both domain novices and the experienced researchers and practitioners. To achieve this goal, the book first covers the foundations of optimization, including problem and algorithm types. Then, well-structured chapters present some of the key studies on ML-based enhancements in the EMâO domain, systematically addressing important aspects. These include learning to understand the problem structure, converge better, diversify better, simultaneously converge and diversify better, and analyze the Pareto Front. In doing so, this book broadly summarizes the literature, beginning with foundational work on innovization (2003) and objective reduction (2006), and extending to the most recently proposed innovized progress operators (2021-23). It also highlights the utility of ML interventions in the search, post-optimality, and decision-making phases pertaining to the use of EMâOAs. Finally, this book shares insightful perspectives on the future potential for ML based enhancements in the EMâOA domain.To aid readers, the book includes working codes for the developed algorithms. This book will not only strengthen this emergent theme but also encourage ML researchers to develop more efficient and scalable methods that cater to the requirements of the EMâOA domain. It serves as an inspiration for further research and applications at the synergistic intersection of EMâOA and ML domains.
To aid readers, the book includes working codes for the developed algorithms. This book will not only strengthen this emergent theme but also encourage ML researchers to develop more efficient and scalable methods that cater to the requirements of the EMâOA domain. It serves as an inspiration for further research and applications at the synergistic intersection of EMâOA and ML domains.
To aid readers, the book includes working codes for the developed algorithms. This book will not only strengthen this emergent theme but also encourage ML researchers to develop more efficient and scalable methods that cater to the requirements of the EMâOA domain. It serves as an inspiration for further research and applications at the synergistic intersection of EMâOA and ML domains.