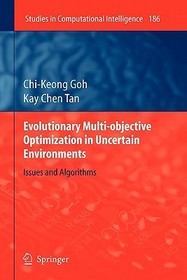
Evolutionary Multi-objective Optimization in Uncertain Environments
Issues and Algorithms
Series: Studies in Computational Intelligence; 186;
- Publisher's listprice EUR 106.99
-
The price is estimated because at the time of ordering we do not know what conversion rates will apply to HUF / product currency when the book arrives. In case HUF is weaker, the price increases slightly, in case HUF is stronger, the price goes lower slightly.
- Discount 20% (cc. 9 077 Ft off)
- Discounted price 36 307 Ft (34 578 Ft + 5% VAT)
45 385 Ft
Availability
Estimated delivery time: In stock at the publisher, but not at Prospero's office. Delivery time approx. 3-5 weeks.
Not in stock at Prospero.
Why don't you give exact delivery time?
Delivery time is estimated on our previous experiences. We give estimations only, because we order from outside Hungary, and the delivery time mainly depends on how quickly the publisher supplies the book. Faster or slower deliveries both happen, but we do our best to supply as quickly as possible.
Product details:
- Edition number Softcover reprint of hardcover 1st ed. 2009
- Publisher Springer
- Date of Publication 28 October 2010
- Number of Volumes 1 pieces, Previously published in hardcover
- ISBN 9783642101137
- Binding Paperback
- No. of pages271 pages
- Size 235x155 mm
- Weight 454 g
- Language English
- Illustrations XI, 271 p. Tables, black & white 0
Categories
Short description:
Evolutionary algorithms are sophisticated search methods that have been found to be very efficient and effective in solving complex real-world multi-objective problems where conventional optimization tools fail to work well. Despite the tremendous amount of work done in the development of these algorithms in the past decade, many researchers assume that the optimization problems are deterministic and uncertainties are rarely examined.
The primary motivation of this book is to provide a comprehensive introduction on the design and application of evolutionary algorithms for multi-objective optimization in the presence of uncertainties. In this book, we hope to expose the readers to a range of optimization issues and concepts, and to encourage a greater degree of appreciation of evolutionary computation techniques and the exploration of new ideas that can better handle uncertainties. "Evolutionary Multi-Objective Optimization in Uncertain Environments: Issues and Algorithms" is intended for a wide readership and will be a valuable reference for engineers, researchers, senior undergraduates and graduate students who are interested in the areas of evolutionary multi-objective optimization and uncertainties.
MoreLong description:
Evolutionary algorithms are sophisticated search methods that have been found to be very efficient and effective in solving complex real-world multi-objective problems where conventional optimization tools fail to work well. Despite the tremendous amount of work done in the development of these algorithms in the past decade, many researchers assume that the optimization problems are deterministic and uncertainties are rarely examined.
The primary motivation of this book is to provide a comprehensive introduction on the design and application of evolutionary algorithms for multi-objective optimization in the presence of uncertainties. In this book, we hope to expose the readers to a range of optimization issues and concepts, and to encourage a greater degree of appreciation of evolutionary computation techniques and the exploration of new ideas that can better handle uncertainties. "Evolutionary Multi-Objective Optimization in Uncertain Environments: Issues and Algorithms" is intended for a wide readership and will be a valuable reference for engineers, researchers, senior undergraduates and graduate students who are interested in the areas of evolutionary multi-objective optimization and uncertainties.
MoreTable of Contents:
I: Evolving Solution Sets in the Presence of Noise.- Noisy Evolutionary Multi-objective Optimization.- Handling Noise in Evolutionary Multi-objective Optimization.- Handling Noise in Evolutionary Neural Network Design.- II: Tracking Dynamic Multi-objective Landscapes.- Dynamic Evolutionary Multi-objective Optimization.- A Coevolutionary Paradigm for Dynamic Multi-Objective Optimization.- III: Evolving Robust Solution Sets.- Robust Evolutionary Multi-objective Optimization.- Evolving Robust Solutions in Multi-Objective Optimization.- Evolving Robust Routes.- Final Thoughts.
More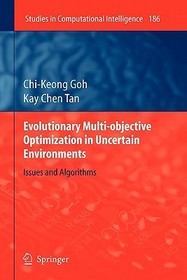
Evolutionary Multi-objective Optimization in Uncertain Environments: Issues and Algorithms
Subcribe now and receive a favourable price.
Subscribe
45 385 HUF