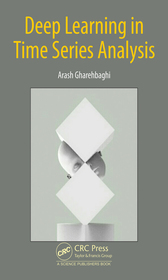
Deep Learning in Time Series Analysis
- Publisher's listprice GBP 130.00
-
The price is estimated because at the time of ordering we do not know what conversion rates will apply to HUF / product currency when the book arrives. In case HUF is weaker, the price increases slightly, in case HUF is stronger, the price goes lower slightly.
- Discount 20% (cc. 13 159 Ft off)
- Discounted price 52 634 Ft (50 128 Ft + 5% VAT)
65 793 Ft
Availability
Estimated delivery time: In stock at the publisher, but not at Prospero's office. Delivery time approx. 3-5 weeks.
Not in stock at Prospero.
Why don't you give exact delivery time?
Delivery time is estimated on our previous experiences. We give estimations only, because we order from outside Hungary, and the delivery time mainly depends on how quickly the publisher supplies the book. Faster or slower deliveries both happen, but we do our best to supply as quickly as possible.
Product details:
- Edition number 1
- Publisher CRC Press
- Date of Publication 7 July 2023
- ISBN 9780367321789
- Binding Hardback
- No. of pages208 pages
- Size 234x156 mm
- Weight 467 g
- Language English
- Illustrations 36 Illustrations, black & white; 17 Illustrations, color; 1 Tables, black & white 518
Categories
Short description:
The concept of deep machine learning is easier to understand by paying attention to the cyclic stochastic time series and a time series whose content is non-stationary not only within the cycles, but also over the cycles as the cycle-to-cycle variations.
MoreLong description:
Deep learning is an important element of artificial intelligence, especially in applications such as image classification in which various architectures of neural network, e.g., convolutional neural networks, have yielded reliable results. This book introduces deep learning for time series analysis, particularly for cyclic time series. It elaborates on the methods employed for time series analysis at the deep level of their architectures. Cyclic time series usually have special traits that can be employed for better classification performance. These are addressed in the book. Processing cyclic time series is also covered herein.
An important factor in classifying stochastic time series is the structural risk associated with the architecture of classification methods. The book addresses and formulates structural risk, and the learning capacity defined for a classification method. These formulations and the mathematical derivations will help the researchers in understanding the methods and even express their methodologies in an objective mathematical way. The book has been designed as a self-learning textbook for the readers with different backgrounds and understanding levels of machine learning, including students, engineers, researchers, and scientists of this domain. The numerous informative illustrations presented by the book will lead the readers to a deep level of understanding about the deep learning methods for time series analysis.
MoreTable of Contents:
PREFACE. I-FUNDAMENTALS OF LEARNING. Introduction to Learning. Learning Theory. Pre-processing and Visualisation. II ESSENTIALS OF TIME SERIES ANALYSIS. Basics of Time Series. Multi-Layer Perceptron (MLP) Neural Networks for Time Series Classification. Dynamic Models for Sequential Data Analysis. III DEEP LEARNING APPROACHES TO TIME SERIES CLASSIFICATION. Clustering for Learning at Deep Level. Deep Time Growing Neural Network. Deep Learning of Cyclic Time Series. Hybrid Method for Cyclic Time Series. Recurrent Neural Networks (RNN). Convolutional Neural Networks. Bibliography.
More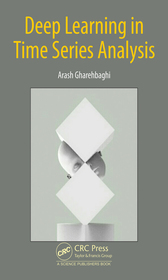
Deep Learning in Time Series Analysis
Subcribe now and receive a favourable price.
Subscribe
65 793 HUF