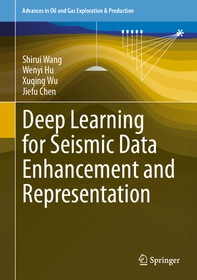
Deep Learning for Seismic Data Enhancement and Representation
Series: Advances in Oil and Gas Exploration & Production;
- Publisher's listprice EUR 181.89
-
The price is estimated because at the time of ordering we do not know what conversion rates will apply to HUF / product currency when the book arrives. In case HUF is weaker, the price increases slightly, in case HUF is stronger, the price goes lower slightly.
- Discount 8% (cc. 6 173 Ft off)
- Discounted price 70 985 Ft (67 604 Ft + 5% VAT)
77 157 Ft
Availability
Estimated delivery time: In stock at the publisher, but not at Prospero's office. Delivery time approx. 3-5 weeks.
Not in stock at Prospero.
Why don't you give exact delivery time?
Delivery time is estimated on our previous experiences. We give estimations only, because we order from outside Hungary, and the delivery time mainly depends on how quickly the publisher supplies the book. Faster or slower deliveries both happen, but we do our best to supply as quickly as possible.
Product details:
- Edition number 2024
- Publisher Springer
- Date of Publication 19 December 2024
- Number of Volumes 1 pieces, Book
- ISBN 9783031757440
- Binding Hardback
- No. of pages152 pages
- Size 254x178 mm
- Language English
- Illustrations 27 Illustrations, black & white; 80 Illustrations, color 672
Categories
Short description:
Seismic imaging is a key component of subsurface exploration, and it depends on a high-quality seismic data acquisition system with effective seismic processing algorithms. Seismic data quality concerns various factors such as acquisition design, environmental constraints, sampling resolution, and noises. The focus of this book is to investigate efficient seismic data representation and signal enhancement solutions by leveraging the powerful feature engineering capability of deep learning.
The book delves into seismic data representation and enhancement issues, ranging from seismic acquisition design to subsequent quality improvement and compression technologies. Given the challenges of obtaining suitable labeled training datasets for seismic data processing problems, we concentrate on exploring deep learning approaches that eliminate the need for labels. We combined novel deep learning techniques with conventional seismic data processing methods, and construct networks and frameworks tailored for seismic data processing. The editors and authors of this book come from both academia and industry with hands-on experiences in seismic data processing and imaging.
MoreLong description:
Seismic imaging is a key component of subsurface exploration, and it depends on a high-quality seismic data acquisition system with effective seismic processing algorithms. Seismic data quality concerns various factors such as acquisition design, environmental constraints, sampling resolution, and noises. The focus of this book is to investigate efficient seismic data representation and signal enhancement solutions by leveraging the powerful feature engineering capability of deep learning.
The book delves into seismic data representation and enhancement issues, ranging from seismic acquisition design to subsequent quality improvement and compression technologies. Given the challenges of obtaining suitable labeled training datasets for seismic data processing problems, we concentrate on exploring deep learning approaches that eliminate the need for labels. We combined novel deep learning techniques with conventional seismic data processing methods, and construct networks and frameworks tailored for seismic data processing. The editors and authors of this book come from both academia and industry with hands-on experiences in seismic data processing and imaging.
MoreTable of Contents:
Chapter 1: Introduction.- Chapter 2: Full Waveform Inversion With Low-Frequency Extrapolation.- 3: Deep Learning For Seismic Deblending.- Chapter 4: Blind-Trace Network For Self-Supervised Seismic Data Interpolation.- Chapter 5: Self-Supervised Learning For Anti-Aliased Seismic Data Interpolation Using Dip Information.- Chapter 6:Deep Learning For Seismic Data Compression.- Chapter 7: Conclusion.
More