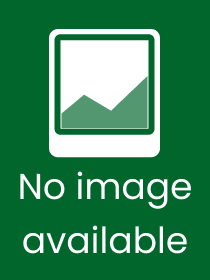
Product details:
ISBN13: | 9780443223419 |
ISBN10: | 0443223416 |
Binding: | Paperback |
No. of pages: | 152 pages |
Language: | English |
700 |
Category:
Bio-Inspired Strategies for Modeling and Detection in Diabetes Mellitus Treatment
Publisher: Morgan Kaufmann
Date of Publication: 3 May 2024
Normal price:
Publisher's listprice:
EUR 170.00
EUR 170.00
Your price:
56 120 (53 448 HUF + 5% VAT )
discount is: 20% (approx 14 030 HUF off)
Discount is valid until: 30 June 2024
The discount is only available for 'Alert of Favourite Topics' newsletter recipients.
Click here to subscribe.
Click here to subscribe.
Availability:
Not yet published.
Long description:
Bio-Inspired Strategies for Modeling and Detection in Diabetes Mellitus Treatment focuses on bioinspired techniques such as modeling to generate control algorithms for the treatment of diabetes mellitus. The book addresses the identification of diabetes mellitus using a high-order recurrent neural network trained by an extended Kalman filter. The authors also describe the use of metaheuristic algorithms for the parametric identification of compartmental models of diabetes mellitus widely used in research works such as the Sorensen model and the Dallaman model. In addition, the book addresses the modeling of time series for the prediction of risk scenarios such as hyperglycaemia and hypoglycaemia using deep neural networks. The detection of diabetes mellitus in the early stages or when current diagnostic techniques cannot detect glucose intolerance or prediabetes is proposed, carried out by means of deep neural networks present in the literature. Readers will find leading-edge research in diabetes identification based on discrete high-order neural networks trained with an extended Kalman filter; parametric identification of compartmental models used to describe diabetes mellitus; modeling of data obtained by continuous glucose-monitoring sensors for the prediction of risk scenarios such as hyperglycaemia and hypoglycaemia; and screening for glucose intolerance using glucose-tolerance test data and deep neural networks. Application of the proposed approaches is illustrated via simulation and real-time implementations for modeling, prediction, and classification.
- Addresses the online identification of diabetes mellitus using a high-order recurrent neural network trained online by an extended Kalman filter.
- Covers parametric identification of compartmental models used to describe diabetes mellitus.
- Provides modeling of data obtained by continuous glucose-monitoring sensors for the prediction of risk scenarios such as hyperglycaemia and hypoglycaemia.
Table of Contents:
1. Introduction
2. Problem statement
3. Mathematical preliminaries
4. Parameter estimation for glucose-insulin dynamics
5. Neural model for glucose-insulin dynamics
6. Multistep predictor applied to T1DM patients
7. Classification and detection of diabetes mellitus and glucose intolerance
8. Conclusion
2. Problem statement
3. Mathematical preliminaries
4. Parameter estimation for glucose-insulin dynamics
5. Neural model for glucose-insulin dynamics
6. Multistep predictor applied to T1DM patients
7. Classification and detection of diabetes mellitus and glucose intolerance
8. Conclusion