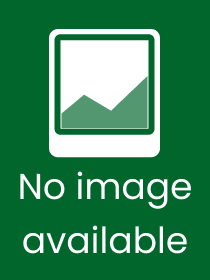
A First Course in Statistical Learning
With Data Examples and Python Code
Series: Statistics and Computing;
- Publisher's listprice EUR 96.29
-
The price is estimated because at the time of ordering we do not know what conversion rates will apply to HUF / product currency when the book arrives. In case HUF is weaker, the price increases slightly, in case HUF is stronger, the price goes lower slightly.
- Discount 8% (cc. 3 268 Ft off)
- Discounted price 37 578 Ft (35 789 Ft + 5% VAT)
40 846 Ft
Availability
Estimated delivery time: In stock at the publisher, but not at Prospero's office. Delivery time approx. 3-5 weeks.
Not in stock at Prospero.
Why don't you give exact delivery time?
Delivery time is estimated on our previous experiences. We give estimations only, because we order from outside Hungary, and the delivery time mainly depends on how quickly the publisher supplies the book. Faster or slower deliveries both happen, but we do our best to supply as quickly as possible.
Product details:
- Edition number 1st ed. 2024
- Publisher Springer
- Date of Publication 26 February 2025
- Number of Volumes 1 pieces, Book
- ISBN 9783031302756
- Binding Hardback
- No. of pages282 pages
- Size 235x155 mm
- Language English
- Illustrations 13 Illustrations, black & white; 714 Illustrations, color 691
Categories
Short description:
This textbook introduces the fundamental concepts and methods of statistical learning. It uses Python and provides a unique approach by blending theory, data examples, software code, and exercises from beginning to end for a profound yet practical introduction to statistical learning.
The book consists of three parts: The first one presents data in the framework of probability theory, exploratory data analysis, and unsupervised learning. The second part on inferential data analysis covers linear and logistic regression and regularization. The last part studies machine learning with a focus on support-vector machines and deep learning. Each chapter is based on a dataset, which can be downloaded from the book's homepage.
In addition, the book has the following features:
- A careful selection of topics ensures rapid progress.
- An opening question at the beginning of each chapter leads the reader through the topic.
- Expositions are rigorous yet based on elementary mathematics.
- More than two hundred exercises help digest the material.
- A crisp discussion section at the end of each chapter summarizes the key concepts and highlights practical implications.
- Numerous suggestions for further reading guide the reader in finding additional information.
This book is for everyone who wants to understand and apply concepts and methods of statistical learning. Typical readers are graduate and advanced undergraduate students in data-intensive fields such as computer science, biology, psychology, business, and engineering, and graduates preparing for their job interviews.
MoreLong description:
This textbook introduces the fundamental concepts and methods of statistical learning. It uses Python and provides a unique approach by blending theory, data examples, software code, and exercises from beginning to end for a profound yet practical introduction to statistical learning.
The book consists of three parts: The first one presents data in the framework of probability theory, exploratory data analysis, and unsupervised learning. The second part on inferential data analysis covers linear and logistic regression and regularization. The last part studies machine learning with a focus on support-vector machines and deep learning. Each chapter is based on a dataset, which can be downloaded from the book's homepage.
In addition, the book has the following features:
- A careful selection of topics ensures rapid progress.
- An opening question at the beginning of each chapter leads the reader through the topic.
- Expositions are rigorous yet based on elementary mathematics.
- More than two hundred exercises help digest the material.
- A crisp discussion section at the end of each chapter summarizes the key concepts and highlights practical implications.
- Numerous suggestions for further reading guide the reader in finding additional information.
This book is for everyone who wants to understand and apply concepts and methods of statistical learning. Typical readers are graduate and advanced undergraduate students in data-intensive fields such as computer science, biology, psychology, business, and engineering, and graduates preparing for their job interviews.
More
Table of Contents:
Part I: Data.- Chapter 1: Fundamentals of Data.- Chapter 2: Exploratory Data Analysis.- Chapter 3: Unsupervised Learning.- Part II: Inferential Data Analyses.- Chapter 4: Linear Regression.- Chapter 5: Logistic Regression.- Chapter 6: Regularization.- Part III: Machine Learning.- Chapter 7: Support-Vector Machines.- Chapter 8: Deep Learning.