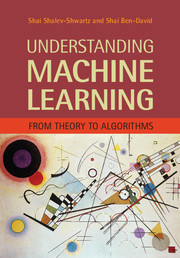
Product details:
ISBN13: | 9781107057135 |
ISBN10: | 1107057132 |
Binding: | Hardback |
No. of pages: | 410 pages |
Size: | 260x183x28 mm |
Weight: | 910 g |
Language: | English |
Illustrations: | 47 b/w illus. 123 exercises |
660 |
Category:
Understanding Machine Learning
From Theory to Algorithms
Publisher: Cambridge University Press
Date of Publication: 19 May 2014
Normal price:
Publisher's listprice:
GBP 50.99
GBP 50.99
Your price:
22 165 (21 110 HUF + 5% VAT )
discount is: 10% (approx 2 463 HUF off)
The discount is only available for 'Alert of Favourite Topics' newsletter recipients.
Click here to subscribe.
Click here to subscribe.
Availability:
Estimated delivery time: In stock at the publisher, but not at Prospero's office. Delivery time approx. 3-5 weeks.
Not in stock at Prospero.
Can't you provide more accurate information?
Not in stock at Prospero.
Short description:
Introduces machine learning and its algorithmic paradigms, explaining the principles behind automated learning approaches and the considerations underlying their usage.
Long description:
Machine learning is one of the fastest growing areas of computer science, with far-reaching applications. The aim of this textbook is to introduce machine learning, and the algorithmic paradigms it offers, in a principled way. The book provides a theoretical account of the fundamentals underlying machine learning and the mathematical derivations that transform these principles into practical algorithms. Following a presentation of the basics, the book covers a wide array of central topics unaddressed by previous textbooks. These include a discussion of the computational complexity of learning and the concepts of convexity and stability; important algorithmic paradigms including stochastic gradient descent, neural networks, and structured output learning; and emerging theoretical concepts such as the PAC-Bayes approach and compression-based bounds. Designed for advanced undergraduates or beginning graduates, the text makes the fundamentals and algorithms of machine learning accessible to students and non-expert readers in statistics, computer science, mathematics and engineering.
'This elegant book covers both rigorous theory and practical methods of machine learning. This makes it a rather unique resource, ideal for all those who want to understand how to find structure in data.' Bernhard Sch&&&246;lkopf, Max Planck Institute for Intelligent Systems, Germany
'This elegant book covers both rigorous theory and practical methods of machine learning. This makes it a rather unique resource, ideal for all those who want to understand how to find structure in data.' Bernhard Sch&&&246;lkopf, Max Planck Institute for Intelligent Systems, Germany
Table of Contents:
1. Introduction; Part I. Foundations: 2. A gentle start; 3. A formal learning model; 4. Learning via uniform convergence; 5. The bias-complexity trade-off; 6. The VC-dimension; 7. Non-uniform learnability; 8. The runtime of learning; Part II. From Theory to Algorithms: 9. Linear predictors; 10. Boosting; 11. Model selection and validation; 12. Convex learning problems; 13. Regularization and stability; 14. Stochastic gradient descent; 15. Support vector machines; 16. Kernel methods; 17. Multiclass, ranking, and complex prediction problems; 18. Decision trees; 19. Nearest neighbor; 20. Neural networks; Part III. Additional Learning Models: 21. Online learning; 22. Clustering; 23. Dimensionality reduction; 24. Generative models; 25. Feature selection and generation; Part IV. Advanced Theory: 26. Rademacher complexities; 27. Covering numbers; 28. Proof of the fundamental theorem of learning theory; 29. Multiclass learnability; 30. Compression bounds; 31. PAC-Bayes; Appendix A. Technical lemmas; Appendix B. Measure concentration; Appendix C. Linear algebra.