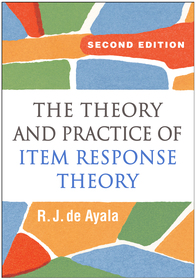
The Theory and Practice of Item Response Theory, Second Edition
- Publisher's listprice GBP 73.99
-
The price is estimated because at the time of ordering we do not know what conversion rates will apply to HUF / product currency when the book arrives. In case HUF is weaker, the price increases slightly, in case HUF is stronger, the price goes lower slightly.
- Discount 20% (cc. 7 489 Ft off)
- Discounted price 29 957 Ft (28 530 Ft + 5% VAT)
37 446 Ft
Availability
Estimated delivery time: In stock at the publisher, but not at Prospero's office. Delivery time approx. 3-5 weeks.
Not in stock at Prospero.
Why don't you give exact delivery time?
Delivery time is estimated on our previous experiences. We give estimations only, because we order from outside Hungary, and the delivery time mainly depends on how quickly the publisher supplies the book. Faster or slower deliveries both happen, but we do our best to supply as quickly as possible.
Product details:
- Edition number 1
- Publisher Guilford Press
- Date of Publication 27 May 2022
- ISBN 9781462547753
- Binding Hardback
- No. of pages643 pages
- Size 254x178 mm
- Weight 1339 g
- Language English 467
Categories
Short description:
Noted for addressing both the "hows" and "whys" of item response theory (IRT), this text has been revised and updated with the latest techniques (multilevel models, mixed models, and more) and software packages. Simple to more complex models are covered in consistently formatted chapters. The book takes the reader from model development through the fit analysis and interpretation phases that would be performed in practice, using common datasets across chapters. Exemplary model applications include free (BIGSTEPS, NOHARM, Facets, R packages) and commercial (BILOG-MG, flexMIRT, SAS, WINMIRA, SPSS, SYSTAT) software packages. The companion website provides data files and online-only appendices.
Long description:
Noted for addressing both the "hows" and "whys" of item response theory (IRT), this text has been revised and updated with the latest techniques (multilevel models, mixed models, and more) and software packages. Simple to more complex models are covered in consistently formatted chapters that build sequentially. The book takes the reader from model development through the fit analysis and interpretation phases that would be performed in practice. To facilitate understanding, common data sets are used across chapters, with the examples worked through for increasingly complex models. Exemplary model applications include free (BIGSTEPS, NOHARM, Facets, R packages) and commercial (BILOG-MG, flexMIRT, SAS, WINMIRA, SPSS, SYSTAT) software packages. The companion website provides data files and online-only appendices.
New to This Edition
*Chapter on multilevel models.
*New material on loglinear models, mixed models, the linear logistic trait model, and fit statistics.
*Many additional worked-through examples.
*Updated guidance on software; now includes R, SAS, and flexMIRT.
"The second edition of the IRT &&&39;bible&&&39; is now even more accessible and useful for psychometricians and educational measurement specialists who are new to IRT or want to upgrade their knowledge of the field. It expands on the first edition in meaningful ways, primarily with regard to the implementation of IRT. Virtually every chapter has been expanded with examples of IRT analyses using R, SAS, and/or flexMIRT. A very helpful new chapter covers multilevel IRT models, and new appendices cover the LLTM and mixture Rasch models and discuss contemporary model fit indices and other recent topics. I have been using the first edition in my advanced measurement class since it was first published and it has been well received by my advanced undergraduates and graduate students; the new material in the second edition makes the book even better. In addition, this book will be very informative to measurement specialists--especially those who are not mathematically sophisticated--so that they can produce instruments that benefit from the enhanced measurement power of IRT.”--David J. Weiss, PhD, Department of Psychology, University of Minnesota
"This is the most comprehensive and accessible text on IRT. De Ayala does a remarkable job of clearly describing fundamental IRT concepts, basic models, and even advanced models. The text&&&39;s explanations do not heavily rely on equations; instead, de Ayala takes a conceptual approach and often utilizes graphs to illustrate key ideas. The second edition is up to date on the most frequently applied models and estimation procedures. It includes applied examples using popular IRT software, including R. I highly recommend this book for graduate-level courses focusing on measurement, psychometrics, and IRT, and as a guide for researchers using IRT."--Ojmarrh Mitchell, PhD, School of Criminology and Criminal Justice, Arizona State University
"I love this book, and find it quite readable. What sets this text apart are its extensive exposition of technical details related to models and estimation and its detailed explanations of concepts. For example, I had never seen an author decompose the partial credit model and show piece-by-piece computation of the probabilities, which de Ayala does very well. This text is a great contribution to the field of IRT that will be invaluable for both class and personal use."--Karen M. Schmidt, PhD, Department of Psychology, University of Virginia
"An excellent treatment of IRT that combines a clear exposition of theoretical concepts with applied examples that are relevant and useful."--Larry R. Price, PhD, College of Education, Texas State University-A must read for practitioners who use item response theory to calibrate test data. It also would serve as a tremendous resource for measurement researchers who daily navigate the circuitous paths of various IRT estimation software programs to analyze and understand their assessment data....Each of the 12 chapters is packed with annotated examples of how to use IRT estimation software and the subsequent output....The author does an excellent job of supplementing explanations of various models with calibration examples and output of multiple data sets using several different IRT calibration software programs including BILOG, MULTILOG, BIGSTEPS, and NOHARM....The book is more practitioner-oriented and applied than previous classic books that provide foundational understanding of IRT models and applications....Would be an excellent text for a graduate level IRT class in which the goal of the course would be to review dichotomous, polytomous, and multidimensional IRT models an how to estimate parameters in the various models using a variety of commercially available software....I would encourage all testing practitioners who work with various IRT models, as well as graduate students who plan to go into the measurement field, to seriously consider this book. It is an excellent resource?.I applaud Dr. de Ayala for all the time and effort he has put into this book. He has clearly done the measurement field a great service. (on the first edition)--Journal of Educational Measurement, 12/21/2010??The main strength of the text is in the descriptions and elaborations of the common IRT models....De Ayala also covers fundamental relationships that exist between models, such as the relationships between the parameters of the nominal response model and the partial credit model. In addition, the chapters contain practical advice for sample sizes commonly used with each model and how to interpret the parameters. De Ayala also presents results as statistical indices and graphics for various examples across different contexts, which allows readers the ability to see how the models work from several different perspectives....Does a good job of introducing common estimation strategies employed in IRT software packages. Especially helpful are the illustrations de Ayala includes with the code from IRT software packages. (on the first edition)--Psychometrika, 12/1/2010
Table of Contents:
Symbols and Acronyms
1. Introduction to Measurement
- Measurement
- Some Measurement Issues
- Item Response Theory
- Classical Test Theory
- Latent Class Analysis
- Summary
2. The One-Parameter Model
- Conceptual Development of the Rasch Model
- The One-Parameter Model
- The One-Parameter Logistic Model and the Rasch Model
- Assumptions Underlying the Model
- An Empirical Data Set: The Mathematics Data Set
- Conceptually Estimating an Individual’s Location
- Some Pragmatic Characteristics of Maximum Likelihood Estimates
- The Standard Error of Estimate and Information
- An Instrument’s Estimation Capacity
- Summary
3. Joint Maximum Likelihood Parameter Estimation
- Joint Maximum Likelihood Estimation
- Indeterminacy of Parameter Estimates
- How Large a Calibration Sample?
- Example: Application of the Rasch Model to the Mathematics Data, JMLE, BIGSTEPS
- Example: Application of the Rasch Model to the Mathematics Data, JMLE, <code>mixRasch</code>
- Validity Evidence
- Summary
4. Marginal Maximum Likelihood Parameter Estimation
- Marginal Maximum Likelihood Estimation
- Estimating an Individual’s Location: Expected A Posteriori
- Example: Application of the Rasch Model to the Mathematics Data, MMLE, BILOG-MG
- Metric Transformation and the Total Characteristic Function
- Example: Application of the Rasch Model to the Mathematics Data, MMLE, <code>mirt</code>
- Summary
5. The Two-Parameter Model
- Conceptual Development of the Two-Parameter Model
- Information for the Two-Parameter Model
- Conceptual Parameter Estimation for the 2PL Model
- How Large a Calibration Sample?
- Metric Transformation, 2PL Model
- Example: Application of the 2PL Model to the Mathematics Data, MMLE, BILOG-MG
- Fit Assessment: An Alternative Approach for Assessing Invariance
- Example: Application of the 2PL Model to the Mathematics Data, MMLE, <code>mirt</code>
- Information and Relative Efficiency
- Summary
6. The Three-Parameter Model
- Conceptual Development of the Three-Parameter Model
- Additional Comments about the Pseudo-Guessing Parameter,<code> X&&&11388;</code>
- Conceptual Parameter Estimation for the 3PL Model
- How Large a Calibration Sample?
- Assessing Conditional Independence
- Example: Application of the 3PL Model to the Mathematics Data, MMLE, BILOG-MG
- Fit Assessment: Conditional Independence Assessment
- Fit Assessment: Model Comparison
- Example: Application of the 3PL Model to the Mathematics Data, MMLE, <code>mirt</code>
- Assessing Person Fit: Appropriateness Measurement
- Information for the Three-Parameter Model
- Metric Transformation, 3PL Model
- Handling Missing Responses
- Issues to Consider in Selecting among the 1PL, 2PL, and 3PL Models
- Summary
7. Rasch Models for Ordered Polytomous Data
- Conceptual Development of the Partial Credit Model
- Conceptual Parameter Estimation of the PC Model
- Example: Application of the PC Model to a Reasoning Ability Instrument, MMLE, flexMIRT
- Example: Application of the PC Model to a Reasoning Ability Instrument, MMLE, <code>mirt</code>
- The Rating Scale Model
- Conceptual Parameter Estimation of the RS Model
- Example: Application of the RS Model to an Attitudes Towards Condoms Scale, JMLE, BIGSTEPS
- Example: Application of the PC Model to an Attitudes Towards Condoms Scale, JMLE, <code>mixRasch</code>
- How Large a Calibration Sample?
- Information for the PC and RS Models
- Metric Transformation, PC and RS Models
- Summary
8. Non-Rasch Models for Ordered Polytomous Data
- The Generalized Partial Credit Model
- Example: Application of the GPC Model to a Reasoning Ability Instrument, MMLE, flexMIRT
- Example: Application of the GPC Model to a Reasoning Ability Instrument, MMLE, <code>mirt</code>
- Conceptual Development of the Graded Response Model
- How Large a Calibration Sample?
- Information for Graded Data
- Metric Transformation, GPC and GR Models
- Example: Application of the GR Model to an Attitudes Towards Condoms Scale, MMLE, flexMIRT
- Example: Application of the GR Model to an Attitudes Towards Condoms Scale, MMLE, <code>mirt</code>
- Conceptual Development of the Continuous Response Model
- Summary
9. Models for Nominal Polytomous Data
- Conceptual Development of the Nominal Response Model
- Information for the NR Model
- Metric Transformation, NR Model
- Conceptual Development of the Multiple-Choice Model
- How Large a Calibration Sample?
- Example: Application of the NR Model to a General Science Test, MMLE, <code>mirt</code>
- Summary
10. Models for Multidimensional Data
- Conceptual Development of a Multidimensional IRT Model
- Multidimensional Item Location and Discrimination
- Item Vectors and Vector Graphs
- The Multidimensional Three-Parameter Logistic Model
- Assumptions of the MIRT Model
- Estimation of the M2PL Model
- Information for the M2PL Model
- Indeterminacy in MIRT
- Metric Transformation, M2PL Model
- Example: Calibration of interpersonal engagement instrument, M2PL Model,<code> sirt.noharam</code>
- Obtaining Person Location Estimates
- Example: Calibration of interpersonal engagement instrument, M2PL Model, <code>mirt</code>
- Example: Calibration of interpersonal engagement instrument, M2PL Model, flexMIRT
- Summary
11. Linking and Equating
- Equating Defined
- Equating: Data Collection Phase
- Equating: Transformation Phase
- Example: Application of the Total Characteristic Function Equating Method, EQUATE
- Example: Application of the Total Characteristic Function Equating Method, <code>SNSequate</code>
- Example: Fixed-item and Concurrent Calibration Equating
- Summary
12. Differential Item Functioning
- Differential Item Functioning and Item Bias
- Mantel–Haenszel Chi-Square
- The TSW Likelihood Ratio Test
- Logistic Regression
- Example: DIF Analysis of vocabulary test, SAS CMH
- Example: DIF Analysis of vocabulary test, <code>mantelhaen.test </code>and <code>difR</code>
- Example: DIF Analysis of vocabulary test, SAS <code>proc logistic</code>
- Example: DIF Analysis of vocabulary test, <code>glm </code>and <code>difR</code>
- Summary
13. Multilevel IRT Models
- Multilevel IRT–Two Levels
- Example: Equivalence of the Rasch model and its Multilevel Model Parameterization, <code>proc glimmix</code>
- Example: Rasch model estimation, <code>lme4</code>
- Person-Level Predictors for Items
- Example: Person-Level Predictors for Items–DIF Analysis, <code>proc glimmix</code>
- Example: Person-Level Predictors for Items–DIF Analysis, <code>lme4</code>
- Person-Level Predictors for Respondents
- Example: Person-Level Predictors for Respondents–Nutrition Literacy,<code> proc glimmix</code>
- Example: Person-Level Predictors for Respondents, <code>lme4</code>
- Item-Level Predictors for Items
- Example: Item-Level Predictors for Items - Nutrition Literacy,<code> proc glimmix</code>
- Example: Item-Level Predictors
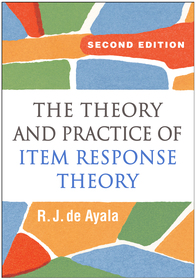
The Theory and Practice of Item Response Theory, Second Edition
Subcribe now and receive a favourable price.
Subscribe
37 446 HUF
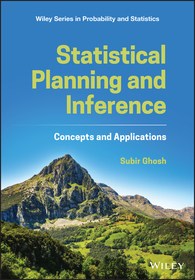
Statistical Planning and Inference ? Concepts and Applications: Concepts and Applications
Subcribe now and receive a favourable price.
Subscribe
35 401 HUF
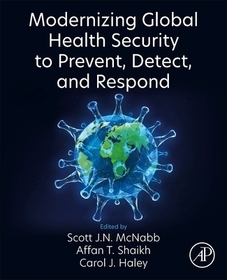
Modernizing Global Health Security to Prevent, Detect, and Respond
Subcribe now and receive a favourable price.
Subscribe
46 237 HUF
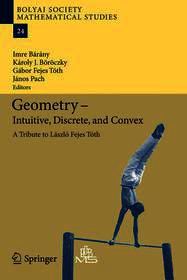
Geometry - Intuitive, Discrete, and Convex: A Tribute to László Fejes Tóth
Subcribe now and receive a favourable price.
Subscribe
22 690 HUF