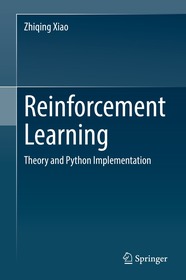
ISBN13: | 9789811949326 |
ISBN10: | 9811949328 |
Binding: | Hardback |
No. of pages: | 593 pages |
Size: | 235x155 mm |
Language: | English |
Illustrations: | 1 Illustrations, black & white; 60 Illustrations, color |
700 |
Probability and mathematical statistics
System analysis, system planning
Operating systems and graphical user interfaces
Artificial Intelligence
Probability and mathematical statistics (charity campaign)
System analysis, system planning (charity campaign)
Operating systems and graphical user interfaces (charity campaign)
Artificial Intelligence (charity campaign)
Reinforcement Learning
EUR 80.24
Click here to subscribe.
Reinforcement Learning: Theory and Python Implementation is a tutorial book on reinforcement learning, with explanations of both theory and applications. Starting from a uniform mathematical framework, this book derives the theory of modern reinforcement learning in a systematic way and introduces all mainstream reinforcement learning algorithms including both classical reinforcement learning algorithms such as eligibility trace and deep reinforcement learning algorithms such as PPO, SAC, and MuZero. Every chapter is accompanied by high-quality implementations based on the latest version of Python packages such as Gym, and the implementations of deep reinforcement learning algorithms are all with both TensorFlow 2 and PyTorch 1. All codes can be found on GitHub along with their results and are runnable on a conventional laptop with either Windows, macOS, or Linux.
This book is intended for readers who want to learn reinforcement learning systematically and apply reinforcement learning to practical applications. It is also ideal to academical researchers who seek theoretical foundation or algorithm enhancement in their cutting-edge AI research.
Reinforcement Learning: Theory and Python Implementation is a tutorial book on reinforcement learning, with explanations of both theory and applications. Starting from a uniform mathematical framework, this book derives the theory of modern reinforcement learning in a systematic way and introduces all mainstream reinforcement learning algorithms including both classical reinforcement learning algorithms such as eligibility trace and deep reinforcement learning algorithms such as PPO, SAC, and MuZero. Every chapter is accompanied by high-quality implementations based on the latest version of Python packages such as Gym, and the implementations of deep reinforcement learning algorithms are all with both TensorFlow 2 and PyTorch 1. All codes can be found on GitHub along with their results and are runnable on a conventional laptop with either Windows, macOS, or Linux.
This book is intended for readers who want to learn reinforcement learning systematically and applyreinforcement learning to practical applications. It is also ideal to academical researchers who seek theoretical foundation or algorithm enhancement in their cutting-edge AI research.