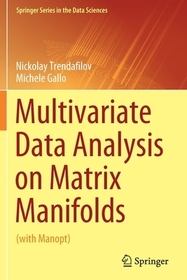
Multivariate Data Analysis on Matrix Manifolds
(with Manopt)
Series: Springer Series in the Data Sciences;
- Publisher's listprice EUR 53.49
-
The price is estimated because at the time of ordering we do not know what conversion rates will apply to HUF / product currency when the book arrives. In case HUF is weaker, the price increases slightly, in case HUF is stronger, the price goes lower slightly.
- Discount 8% (cc. 1 815 Ft off)
- Discounted price 20 874 Ft (19 880 Ft + 5% VAT)
22 690 Ft
Availability
Estimated delivery time: In stock at the publisher, but not at Prospero's office. Delivery time approx. 3-5 weeks.
Not in stock at Prospero.
Why don't you give exact delivery time?
Delivery time is estimated on our previous experiences. We give estimations only, because we order from outside Hungary, and the delivery time mainly depends on how quickly the publisher supplies the book. Faster or slower deliveries both happen, but we do our best to supply as quickly as possible.
Product details:
- Edition number 1st ed. 2021
- Publisher Springer
- Date of Publication 16 September 2022
- Number of Volumes 1 pieces, Book
- ISBN 9783030769765
- Binding Paperback
- No. of pages450 pages
- Size 235x155 mm
- Weight 718 g
- Language English
- Illustrations 1 Illustrations, black & white; 5 Illustrations, color 446
Categories
Short description:
This graduate-level textbook aims to give a unified presentation and solution of several commonly used techniques for multivariate data analysis (MDA). Unlike similar texts, it treats the MDA problems as optimization problems on matrix manifolds defined by the MDA model parameters, allowing them to be solved using (free) optimization software Manopt. The book includes numerous in-text examples as well as Manopt codes and software guides, which can be applied directly or used as templates for solving similar and new problems. The first two chapters provide an overview and essential background for studying MDA, giving basic information and notations. Next, it considers several sets of matrices routinely used in MDA as parameter spaces, along with their basic topological properties. A brief introduction to matrix (Riemannian) manifolds and optimization methods on them with Manopt complete the MDA prerequisite. The remaining chapters study individual MDA techniques in depth. The number ofexercises complement the main text with additional information and occasionally involve open and/or challenging research questions. Suitable fields include computational statistics, data analysis, data mining and data science, as well as theoretical computer science, machine learning and optimization. It is assumed that the readers have some familiarity with MDA and some experience with matrix analysis, computing, and optimization.
MoreLong description:
This graduate-level textbook aims to give a unified presentation and solution of several commonly used techniques for multivariate data analysis (MDA). Unlike similar texts, it treats the MDA problems as optimization problems on matrix manifolds defined by the MDA model parameters, allowing them to be solved using (free) optimization software Manopt. The book includes numerous in-text examples as well as Manopt codes and software guides, which can be applied directly or used as templates for solving similar and new problems. The first two chapters provide an overview and essential background for studying MDA, giving basic information and notations. Next, it considers several sets of matrices routinely used in MDA as parameter spaces, along with their basic topological properties. A brief introduction to matrix (Riemannian) manifolds and optimization methods on them with Manopt complete the MDA prerequisite. The remaining chapters study individual MDA techniques in depth. The number ofexercises complement the main text with additional information and occasionally involve open and/or challenging research questions. Suitable fields include computational statistics, data analysis, data mining and data science, as well as theoretical computer science, machine learning and optimization. It is assumed that the readers have some familiarity with MDA and some experience with matrix analysis, computing, and optimization.
MoreTable of Contents:
Introduction.- Matrix analysis and differentiation.- Matrix manifolds in MDA.- Principal component analysis (PCA).- Factor analysis (FA).- Procrustes analysis (PA).- Linear discriminant analysis (LDA).- Canonical correlation analysis (CCA).- Common principal components (CPC).- Metric multidimensional scaling (MDS) and related methods.- Data analysis on simplexes.
More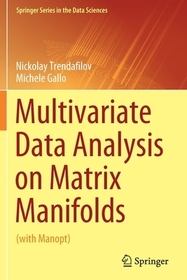
Multivariate Data Analysis on Matrix Manifolds: (with Manopt)
Subcribe now and receive a favourable price.
Subscribe
22 690 HUF