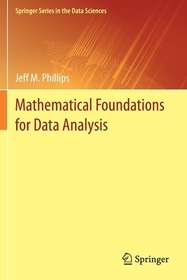
Mathematical Foundations for Data Analysis
Series: Springer Series in the Data Sciences;
- Publisher's listprice EUR 58.84
-
The price is estimated because at the time of ordering we do not know what conversion rates will apply to HUF / product currency when the book arrives. In case HUF is weaker, the price increases slightly, in case HUF is stronger, the price goes lower slightly.
- Discount 8% (cc. 1 997 Ft off)
- Discounted price 22 963 Ft (21 869 Ft + 5% VAT)
24 959 Ft
Availability
Estimated delivery time: In stock at the publisher, but not at Prospero's office. Delivery time approx. 3-5 weeks.
Not in stock at Prospero.
Why don't you give exact delivery time?
Delivery time is estimated on our previous experiences. We give estimations only, because we order from outside Hungary, and the delivery time mainly depends on how quickly the publisher supplies the book. Faster or slower deliveries both happen, but we do our best to supply as quickly as possible.
Product details:
- Edition number 1st ed. 2021
- Publisher Springer
- Date of Publication 31 March 2022
- Number of Volumes 1 pieces, Book
- ISBN 9783030623432
- Binding Paperback
- No. of pages287 pages
- Size 235x155 mm
- Weight 474 g
- Language English
- Illustrations 1 Illustrations, black & white; 108 Illustrations, color 300
Categories
Short description:
This textbook, suitable for an early undergraduate up to a graduate course, provides an overview of many basic principles and techniques needed for modern data analysis. In particular, this book was designed and written as preparation for students planning to take rigorous Machine Learning and Data Mining courses. It introduces key conceptual tools necessary for data analysis, including concentration of measure and PAC bounds, cross validation, gradient descent, and principal component analysis. It also surveys basic techniques in supervised (regression and classification) and unsupervised learning (dimensionality reduction and clustering) through an accessible, simplified presentation. Students are recommended to have some background in calculus, probability, and linear algebra. Some familiarity with programming and algorithms is useful to understand advanced topics on computational techniques.
MoreLong description:
This textbook, suitable for an early undergraduate up to a graduate course, provides an overview of many basic principles and techniques needed for modern data analysis. In particular, this book was designed and written as preparation for students planning to take rigorous Machine Learning and Data Mining courses. It introduces key conceptual tools necessary for data analysis, including concentration of measure and PAC bounds, cross validation, gradient descent, and principal component analysis. It also surveys basic techniques in supervised (regression and classification) and unsupervised learning (dimensionality reduction and clustering) through an accessible, simplified presentation. Students are recommended to have some background in calculus, probability, and linear algebra. Some familiarity with programming and algorithms is useful to understand advanced topics on computational techniques.
?This is certainly a timely book with large potential impact and appeal. ? the book is therewith accessible to a broad scientific audience including undergraduate students. ? Mathematical Foundations for Data Analysis provides a comprehensive exploration of the mathematics relevant to modern data science topics, with a target audience that is looking for an intuitive and accessible presentation rather than a deep dive into mathematical intricacies.? (Aretha L. Teckentrup, SIAM Review, Vol. 65 (1), March, 2023)
Table of Contents:
Probability review.- Convergence and sampling.- Linear algebra review.- Distances and nearest neighbors.- Linear Regression.- Gradient descent.- Dimensionality reduction.- Clustering.- Classification.- Graph structured data.- Big data and sketching.
More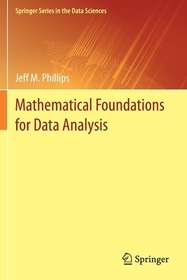
Mathematical Foundations for Data Analysis
Subcribe now and receive a favourable price.
Subscribe
24 959 HUF