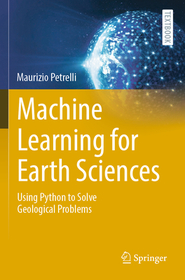
Machine Learning for Earth Sciences
Using Python to Solve Geological Problems
Series: Springer Textbooks in Earth Sciences, Geography and Environment;
- Publisher's listprice EUR 64.19
-
The price is estimated because at the time of ordering we do not know what conversion rates will apply to HUF / product currency when the book arrives. In case HUF is weaker, the price increases slightly, in case HUF is stronger, the price goes lower slightly.
- Discount 8% (cc. 2 178 Ft off)
- Discounted price 25 050 Ft (23 857 Ft + 5% VAT)
27 229 Ft
Availability
Estimated delivery time: In stock at the publisher, but not at Prospero's office. Delivery time approx. 3-5 weeks.
Not in stock at Prospero.
Why don't you give exact delivery time?
Delivery time is estimated on our previous experiences. We give estimations only, because we order from outside Hungary, and the delivery time mainly depends on how quickly the publisher supplies the book. Faster or slower deliveries both happen, but we do our best to supply as quickly as possible.
Product details:
- Edition number 2023
- Publisher Springer
- Date of Publication 24 September 2024
- Number of Volumes 1 pieces, Book
- ISBN 9783031351167
- Binding Paperback
- No. of pages209 pages
- Size 235x155 mm
- Language English
- Illustrations 3 Illustrations, black & white; 99 Illustrations, color 648
Categories
Short description:
This textbook introduces the reader to Machine Learning (ML) applications in Earth Sciences. In detail, it starts by describing the basics of machine learning and its potentials in Earth Sciences to solve geological problems. It describes the main Python tools devoted to ML, the typical workflow of ML applications in Earth Sciences, and proceeds with reporting how ML algorithms work. The book provides many examples of ML application to Earth Sciences problems in many fields, such as the clustering and dimensionality reduction in petro-volcanological studies, the clustering of multi-spectral data, well-log data facies classification, and machine learning regression in petrology. Also, the book introduces the basics of parallel computing and how to scale ML models in the cloud. The book is devoted to Earth Scientists, at any level, from students to academics and professionals.
MoreLong description:
This textbook introduces the reader to Machine Learning (ML) applications in Earth Sciences. In detail, it starts by describing the basics of machine learning and its potentials in Earth Sciences to solve geological problems. It describes the main Python tools devoted to ML, the typical workflow of ML applications in Earth Sciences, and proceeds with reporting how ML algorithms work. The book provides many examples of ML application to Earth Sciences problems in many fields, such as the clustering and dimensionality reduction in petro-volcanological studies, the clustering of multi-spectral data, well-log data facies classification, and machine learning regression in petrology. Also, the book introduces the basics of parallel computing and how to scale ML models in the cloud. The book is devoted to Earth Scientists, at any level, from students to academics and professionals.
?This book is essential for anyone planning to apply machine learning to earth science data (including multispectral and hyperspectral imaging). For maximum benefit, the reader should treat it as both an extensive tutorial as well as a bibliography: be prepared to code along with the examples and to look up the references.? (Creed Jones, Computing Reviews, January 1, 2024) More
Table of Contents:
Part 1: Basic Concepts of Machine Learning for Earth Scientists.- Chapter 1. Introduction to Machine Learning.- Chapter 2. Setting Up your Python Environments for Machine Learning.- Chapter 3. Machine Learning Workflow.- Part 2: Unsupervised Learning.- Chapter 4. Unsupervised Machine Learning Methods.- Chapter 5. Clustering and Dimensionality Reduction in Petrology.- Chapter 6. Clustering of Multi-Spectral Data.- Part 3: Supervised Learning.- Chapter 7. Supervised Machine Learning Methods.- Chapter 8. Classification of Well Log Data Facies by Machine Learning.- Chapter 9. Machine Learning Regression in Petrology.- Part 4: Scaling Machine Learning Models.- Chapter 10. Parallel Computing and Scaling with Dask.- Chapter 11. Scale Your Models in the Cloud.- Part 5: Next Step: Deep Learning.- Chapter 12. Introduction to Deep Learning.
More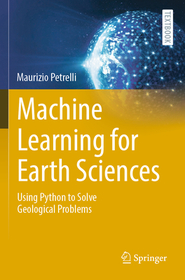
Machine Learning for Earth Sciences: Using Python to Solve Geological Problems
Subcribe now and receive a favourable price.
Subscribe
27 229 HUF