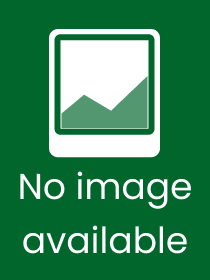
Implementation and Interpretation of Machine and Deep Learning to Applied Subsurface Geological Problems
Prediction Models Exploiting Well-Log Information
- Publisher's listprice EUR 153.99
-
The price is estimated because at the time of ordering we do not know what conversion rates will apply to HUF / product currency when the book arrives. In case HUF is weaker, the price increases slightly, in case HUF is stronger, the price goes lower slightly.
- Discount 10% (cc. 6 532 Ft off)
- Discounted price 58 789 Ft (55 990 Ft + 5% VAT)
65 322 Ft
Availability
printed on demand
Why don't you give exact delivery time?
Delivery time is estimated on our previous experiences. We give estimations only, because we order from outside Hungary, and the delivery time mainly depends on how quickly the publisher supplies the book. Faster or slower deliveries both happen, but we do our best to supply as quickly as possible.
Product details:
- Publisher Elsevier
- Date of Publication 25 March 2025
- ISBN 9780443265105
- Binding Paperback
- No. of pages442 pages
- Size 235x191 mm
- Weight 450 g
- Language English 698
Categories
Long description:
Implementation and Interpretation of Machine and Deep Learning to Applied Subsurface Geological Problems: Prediction Models Exploiting Well-Log Information explores machine and deep learning models for subsurface geological prediction problems commonly encountered in applied resource evaluation and reservoir characterization tasks. The book provides insights into how the performance of ML/DL models can be optimized-and sparse datasets of input variables enhanced and/or rescaled-to improve prediction performances. A variety of topics are covered, including regression models to estimate total organic carbon from well-log data, predicting brittleness indexes in tight formation sequences, trapping mechanisms in potential sub-surface carbon storage reservoirs, and more.
Each chapter includes its own introduction, summary, and nomenclature sections, along with one or more case studies focused on prediction model implementation related to its topic.
- Addresses common applied geological problems focused on machine and deep learning implementation with case studies
- Considers regression, classification, and clustering machine learning methods and how to optimize and assess their performance, considering suitable error and accuracy metric
- Contrasts the pros and cons of multiple machine and deep learning methods
- Includes techniques to improve the identification of geological carbon capture and storage reservoirs, a key part of many energy transition strategies
Table of Contents:
1. Regression models to estimate total organic carbon (TOC) from well-log data
2. Predicting brittleness indexes in tight formation sequences
3. Classifying lithofacies in clastic, carbonate, and mixed reservoir sequences
4. Permeability and water saturation distributions in complex reservoirs
5. Trapping mechanisms in potential sub-surface carbon storage reservoirs
6. The accurate picking of formation tops in field development wells
7. Assessing formation loss of circulation risks with mud-log datasets
8. Delineating fracture densities and apertures using well-log image data
9. Determining reservoir microfacies using photomicrograph and computed tomography image data
10. Characterizing coal-bed methane reservoirs with well-log datasets