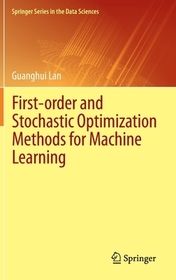
First-order and Stochastic Optimization Methods for Machine Learning
Series: Springer Series in the Data Sciences;
- Publisher's listprice EUR 149.79
-
The price is estimated because at the time of ordering we do not know what conversion rates will apply to HUF / product currency when the book arrives. In case HUF is weaker, the price increases slightly, in case HUF is stronger, the price goes lower slightly.
- Discount 8% (cc. 5 083 Ft off)
- Discounted price 58 457 Ft (55 674 Ft + 5% VAT)
63 540 Ft
Availability
Estimated delivery time: In stock at the publisher, but not at Prospero's office. Delivery time approx. 3-5 weeks.
Not in stock at Prospero.
Why don't you give exact delivery time?
Delivery time is estimated on our previous experiences. We give estimations only, because we order from outside Hungary, and the delivery time mainly depends on how quickly the publisher supplies the book. Faster or slower deliveries both happen, but we do our best to supply as quickly as possible.
Product details:
- Edition number 1st ed. 2020
- Publisher Springer
- Date of Publication 16 May 2020
- Number of Volumes 1 pieces, Book
- ISBN 9783030395674
- Binding Hardback
- No. of pages582 pages
- Size 235x155 mm
- Weight 1051 g
- Language English
- Illustrations 2 Illustrations, black & white; 16 Illustrations, color 112
Categories
Short description:
This book covers not only foundational materials but also the most recent progresses made during the past few years on the area of machine learning algorithms. In spite of the intensive research and development in this area, there does not exist a systematic treatment to introduce the fundamental concepts and recent progresses on machine learning algorithms, especially on those based on stochastic optimization methods, randomized algorithms, nonconvex optimization, distributed and online learning, and projection free methods. This book will benefit the broad audience in the area of machine learning, artificial intelligence and mathematical programming community by presenting these recent developments in a tutorial style, starting from the basic building blocks to the most carefully designed and complicated algorithms for machine learning.
MoreLong description:
This book covers not only foundational materials but also the most recent progresses made during the past few years on the area of machine learning algorithms. In spite of the intensive research and development in this area, there does not exist a systematic treatment to introduce the fundamental concepts and recent progresses on machine learning algorithms, especially on those based on stochastic optimization methods, randomized algorithms, nonconvex optimization, distributed and online learning, and projection free methods. This book will benefit the broad audience in the area of machine learning, artificial intelligence and mathematical programming community by presenting these recent developments in a tutorial style, starting from the basic building blocks to the most carefully designed and complicated algorithms for machine learning.
Table of Contents:
Machine Learning Models.- Convex Optimization Theory.- Deterministic Convex Optimization.- Stochastic Convex Optimization.- Convex Finite-sum and Distributed Optimization.- Nonconvex Optimization.- Projection-free Methods.- Operator Sliding and Decentralized Optimization.
More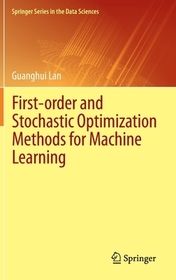
First-order and Stochastic Optimization Methods for Machine Learning
Subcribe now and receive a favourable price.
Subscribe
63 540 HUF