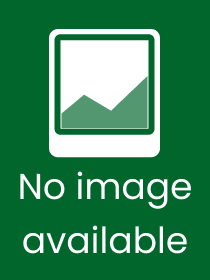
Evolutionary Multi-Task Optimization
Foundations and Methodologies
Series: Machine Learning: Foundations, Methodologies, and Applications;
- Publisher's listprice EUR 181.89
-
The price is estimated because at the time of ordering we do not know what conversion rates will apply to HUF / product currency when the book arrives. In case HUF is weaker, the price increases slightly, in case HUF is stronger, the price goes lower slightly.
- Discount 8% (cc. 6 173 Ft off)
- Discounted price 70 985 Ft (67 604 Ft + 5% VAT)
77 157 Ft
Availability
Not yet published.
Why don't you give exact delivery time?
Delivery time is estimated on our previous experiences. We give estimations only, because we order from outside Hungary, and the delivery time mainly depends on how quickly the publisher supplies the book. Faster or slower deliveries both happen, but we do our best to supply as quickly as possible.
Product details:
- Edition number 1st ed. 2023
- Publisher Springer
- Date of Publication 2 April 2025
- Number of Volumes 1 pieces, Book
- ISBN 9789811956522
- Binding Paperback
- No. of pages219 pages
- Size 235x155 mm
- Language English
- Illustrations 1 Illustrations, black & white 700
Categories
Short description:
Recently, a novel evolutionary search paradigm, Evolutionary Multi-Task (EMT) optimization, has been proposed in the realm of evolutionary computation. In contrast to traditional evolutionary searches, which solve a single task in a single run, evolutionary multi-tasking algorithm conducts searches concurrently on multiple search spaces corresponding to different tasks or optimization problems,each possessing a unique function landscape. By exploiting the latent synergies among distinct problems, the superior search performance of EMT optimization in terms of solution quality and convergence speed has been demonstrated in a variety of continuous, discrete, and hybrid (mixture of continuous and discrete) tasks.
This book discusses the foundations and methodologies of developing evolutionary multi-tasking algorithms for complex optimization, including in domains characterized by factors such as multiple objectives of interest, high-dimensional search spaces and NP-hardness.
MoreLong description:
Recently, a novel evolutionary search paradigm, Evolutionary Multi-Task (EMT) optimization, has been proposed in the realm of evolutionary computation. In contrast to traditional evolutionary searches, which solve a single task in a single run, evolutionary multi-tasking algorithm conducts searches concurrently on multiple search spaces corresponding to different tasks or optimization problems,each possessing a unique function landscape. By exploiting the latent synergies among distinct problems, the superior search performance of EMT optimization in terms of solution quality and convergence speed has been demonstrated in a variety of continuous, discrete, and hybrid (mixture of continuous and discrete) tasks.
This book discusses the foundations and methodologies of developing evolutionary multi-tasking algorithms for complex optimization, including in domains characterized by factors such as multiple objectives of interest, high-dimensional search spaces and NP-hardness.
MoreTable of Contents:
Chapter 1.Introduction.- Chapter 2. Overview and Application-driven Motivations of Evolutionary Multitasking.- Chapter 3.The Multi-factorial Evolutionary Algorithm.- Chapter 4. Multi-factorial Evolutionary Algorithm with Adaptive Knowledge Transfer.- Chapter 5.Explicit Evolutionary Multi-task Optimization Algorithm.- Chapter 6.Evolutionary Multi-task Optimization for Generalized Vehicle Routing Problem With Occasional Drivers.- Chapter 7. Explicit Evolutionary Multi-task Optimization for Capacitated Vehicle Routing Problem.- Chapter 8. Multi-Space Evolutionary Search for Large Scale Single-Objective Optimization.- Chapter 9.Multi-Space Evolutionary Search for Large-scale Multi-Objective Optimization.
More