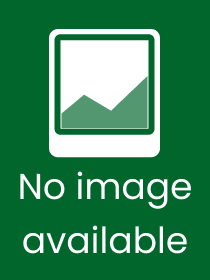
Continual and Reinforcement Learning for Edge AI
Framework, Foundation, and Algorithm Design
Series: Synthesis Lectures on Learning, Networks, and Algorithms;
- Publisher's listprice EUR 42.79
-
The price is estimated because at the time of ordering we do not know what conversion rates will apply to HUF / product currency when the book arrives. In case HUF is weaker, the price increases slightly, in case HUF is stronger, the price goes lower slightly.
- Discount 8% (cc. 1 452 Ft off)
- Discounted price 16 699 Ft (15 904 Ft + 5% VAT)
18 151 Ft
Availability
Not yet published.
Why don't you give exact delivery time?
Delivery time is estimated on our previous experiences. We give estimations only, because we order from outside Hungary, and the delivery time mainly depends on how quickly the publisher supplies the book. Faster or slower deliveries both happen, but we do our best to supply as quickly as possible.
Product details:
- Publisher Springer
- Date of Publication 17 June 2025
- Number of Volumes 1 pieces, Book
- ISBN 9783031843624
- Binding Hardback
- No. of pages274 pages
- Size 240x168 mm
- Language English
- Illustrations 2 Illustrations, black & white; 44 Illustrations, color 700
Categories
Short description:
This book provides a comprehensive introduction to continual and reinforcement learning for edge AI, which investigates how to build an AI agent that can continuously solve new learning tasks and enhance the AI at resource-limited edge devices. The authors introduce readers to practical frameworks and in-depth algorithmic foundations. The book surveys the recent advances in the area, coming from both academic researchers and industry professionals. The authors also present their own research findings on continual and reinforcement learning for edge AI. The book also covers the practical applications of the topic and identifies exciting future research opportunities.
In addition, this book:
- Provides readers with a deep understanding of the topic, including the framework, foundations, and recent advances
- Discusses the applications of continual and reinforcement learning for edge AI, emphasizing its importance
- Familiarizes readers with the current development stage of the topic in order to inspire further research
About the Authors
Hang Wang is a Ph.D. candidate in the Department of Electrical and Computer Engineering at the University of California, Davis. He received his B.E. from the University of Science and Technology of China (USTC).
Sen Lin, Ph.D., is an Assistant Professor in the Department of Computer Science at University of Houston. He received his Ph.D. degree from Arizona State University, M.S. from HKUST and B.E. from Zhejiang University.
Junshan Zhang, Ph.D. is a Professor in the ECE Department at the University of California, Davis. He received his Ph.D. from the School of ECE at Purdue University.
MoreLong description:
This book provides a comprehensive introduction to continual and reinforcement learning for edge AI, which investigates how to build an AI agent that can continuously solve new learning tasks and enhance the AI at resource-limited edge devices. The authors introduce readers to practical frameworks and in-depth algorithmic foundations. The book surveys the recent advances in the area, coming from both academic researchers and industry professionals. The authors also present their own research findings on continual and reinforcement learning for edge AI. The book also covers the practical applications of the topic and identifies exciting future research opportunities.
MoreTable of Contents:
Introduction to Continual and Reinforcement Learning for Edge AI.- Algorithmic and Theoretical Foundations.- Federated Continual Learning.- On-device Continual Learning.- Online Meta-Learning.- Warm-start Reinforcement Learning.- Continual Reinforcement Learning.- Continual and Reinforcement Learning for Edge AI with Pre-trained Large Language Models.
More