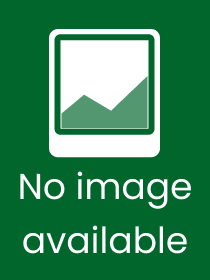
Bayesian Optimization
Theory and Practice Using Python
- Publisher's listprice EUR 64.19
-
The price is estimated because at the time of ordering we do not know what conversion rates will apply to HUF / product currency when the book arrives. In case HUF is weaker, the price increases slightly, in case HUF is stronger, the price goes lower slightly.
- Discount 20% (cc. 5 446 Ft off)
- Discounted price 21 783 Ft (20 746 Ft + 5% VAT)
27 229 Ft
Availability
Estimated delivery time: In stock at the publisher, but not at Prospero's office. Delivery time approx. 3-5 weeks.
Not in stock at Prospero.
Why don't you give exact delivery time?
Delivery time is estimated on our previous experiences. We give estimations only, because we order from outside Hungary, and the delivery time mainly depends on how quickly the publisher supplies the book. Faster or slower deliveries both happen, but we do our best to supply as quickly as possible.
Product details:
- Edition number 1st ed.
- Publisher Apress
- Date of Publication 24 March 2023
- Number of Volumes 1 pieces, Book
- ISBN 9781484290620
- Binding Paperback
- No. of pages234 pages
- Size 254x178 mm
- Weight 485 g
- Language English
- Illustrations 64 Illustrations, black & white; 20 Illustrations, color 590
Categories
Short description:
This book covers the essential theory and implementation of popular Bayesian optimization techniques in an intuitive and well-illustrated manner. The techniques covered in this book will enable you to better tune the hyperparemeters of your machine learning models and learn sample-efficient approaches to global optimization.
The book begins by introducing different Bayesian Optimization (BO) techniques, covering both commonly used tools and advanced topics. It follows a ?develop from scratch? method using Python, and gradually builds up to more advanced libraries such as BoTorch, an open-source project introduced by Facebook recently. Along the way, you?ll see practical implementations of this important discipline along with thorough coverage and straightforward explanations of essential theories. This book intends to bridge the gap between researchers and practitioners, providing both with a comprehensive, easy-to-digest, and useful reference guide.After completing this book, you will have a firm grasp of Bayesian optimization techniques, which you?ll be able to put into practice in your own machine learning models.
- Apply Bayesian Optimization to build better machine learning models
- Understand and research existing and new Bayesian Optimization techniques
- Leverage high-performance libraries such as BoTorch, which offer you the ability to dig into and edit the inner working
- Dig into the inner workings of common optimization algorithms used to guide the search process in Bayesian optimization
Long description:
This book covers the essential theory and implementation of popular Bayesian optimization techniques in an intuitive and well-illustrated manner. The techniques covered in this book will enable you to better tune the hyperparemeters of your machine learning models and learn sample-efficient approaches to global optimization.
The book begins by introducing different Bayesian Optimization (BO) techniques, covering both commonly used tools and advanced topics. It follows a ?develop from scratch? method using Python, and gradually builds up to more advanced libraries such as BoTorch, an open-source project introduced by Facebook recently. Along the way, you?ll see practical implementations of this important discipline along with thorough coverage and straightforward explanations of essential theories. This book intends to bridge the gap between researchers and practitioners, providing both with a comprehensive, easy-to-digest, and useful reference guide. After completingthis book, you will have a firm grasp of Bayesian optimization techniques, which you?ll be able to put into practice in your own machine learning models.
- Apply Bayesian Optimization to build better machine learning models
- Understand and research existing and new Bayesian Optimization techniques
- Leverage high-performance libraries such as BoTorch, which offer you the ability to dig into and edit the inner working
- Dig into the inner workings of common optimization algorithms used to guide the search process in Bayesian optimization
Table of Contents:
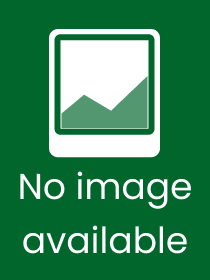
Bayesian Optimization: Theory and Practice Using Python
Subcribe now and receive a favourable price.
Subscribe
27 229 HUF