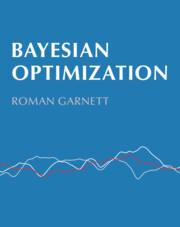
Bayesian Optimization
- Publisher's listprice GBP 44.99
-
The price is estimated because at the time of ordering we do not know what conversion rates will apply to HUF / product currency when the book arrives. In case HUF is weaker, the price increases slightly, in case HUF is stronger, the price goes lower slightly.
- Discount 10% (cc. 2 277 Ft off)
- Discounted price 20 492 Ft (19 517 Ft + 5% VAT)
22 769 Ft
Availability
Estimated delivery time: In stock at the publisher, but not at Prospero's office. Delivery time approx. 3-5 weeks.
Not in stock at Prospero.
Why don't you give exact delivery time?
Delivery time is estimated on our previous experiences. We give estimations only, because we order from outside Hungary, and the delivery time mainly depends on how quickly the publisher supplies the book. Faster or slower deliveries both happen, but we do our best to supply as quickly as possible.
Product details:
- Publisher Cambridge University Press
- Date of Publication 9 February 2023
- ISBN 9781108425780
- Binding Hardback
- No. of pages358 pages
- Size 261x209x22 mm
- Weight 1020 g
- Language English 915
Categories
Short description:
A comprehensive introduction to Bayesian optimization that starts from scratch and carefully develops all the key ideas along the way.
MoreLong description:
Bayesian optimization is a methodology for optimizing expensive objective functions that has proven success in the sciences, engineering, and beyond. This timely text provides a self-contained and comprehensive introduction to the subject, starting from scratch and carefully developing all the key ideas along the way. This bottom-up approach illuminates unifying themes in the design of Bayesian optimization algorithms and builds a solid theoretical foundation for approaching novel situations. The core of the book is divided into three main parts, covering theoretical and practical aspects of Gaussian process modeling, the Bayesian approach to sequential decision making, and the realization and computation of practical and effective optimization policies. Following this foundational material, the book provides an overview of theoretical convergence results, a survey of notable extensions, a comprehensive history of Bayesian optimization, and an extensive annotated bibliography of applications.
MoreTable of Contents:
Notation; 1. Introduction; 2. Gaussian processes; 3. Modeling with Gaussian processes; 4. Model assessment, selection, and averaging; 5. Decision theory for optimization; 6. Utility functions for optimization; 7. Common Bayesian optimization policies; 8. Computing policies with Gaussian processes; 9. Implementation; 10. Theoretical analysis; 11. Extensions and related settings; 12. A brief history of Bayesian optimization; A. The Gaussian distribution; B. Methods for approximate Bayesian inference; C. Gradients; D. Annotated bibliography of applications; References; Index.
More