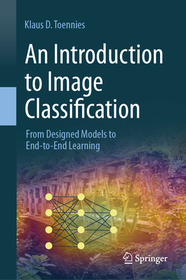
An Introduction to Image Classification
From Designed Models to End-to-End Learning
- Publisher's listprice EUR 74.89
-
The price is estimated because at the time of ordering we do not know what conversion rates will apply to HUF / product currency when the book arrives. In case HUF is weaker, the price increases slightly, in case HUF is stronger, the price goes lower slightly.
- Discount 20% (cc. 6 354 Ft off)
- Discounted price 25 414 Ft (24 204 Ft + 5% VAT)
31 768 Ft
Availability
Estimated delivery time: In stock at the publisher, but not at Prospero's office. Delivery time approx. 3-5 weeks.
Not in stock at Prospero.
Why don't you give exact delivery time?
Delivery time is estimated on our previous experiences. We give estimations only, because we order from outside Hungary, and the delivery time mainly depends on how quickly the publisher supplies the book. Faster or slower deliveries both happen, but we do our best to supply as quickly as possible.
Product details:
- Edition number 1st ed. 2024
- Publisher Springer
- Date of Publication 25 January 2024
- Number of Volumes 1 pieces, Book
- ISBN 9789819978816
- Binding Hardback
- No. of pages290 pages
- Size 235x155 mm
- Weight 629 g
- Language English
- Illustrations 1 Illustrations, black & white 573
Categories
Short description:
Image classification is a critical component in computer vision tasks and has numerous applications. Traditional methods for image classification involve feature extraction and classification in feature space. Current state-of-the-art methods utilize end-to-end learning with deep neural networks, where feature extraction and classification are integrated into the model. Understanding traditional image classification is important because many of its design concepts directly correspond to components of a neural network. This knowledge can help demystify the behavior of these networks, which may seem opaque at first sight.
The book starts from introducing methods for model-driven feature extraction and classification, including basic computer vision techniques for extracting high-level semantics from images. A brief overview of probabilistic classification with generative and discriminative classifiers is then provided. Next, neural networks are presented as a means to learn a classification model directly from labeled sample images, with individual components of the network discussed. The relationships between network components and those of a traditional designed model are explored, and different concepts for regularizing model training are explained. Finally, various methods for analyzing what a network has learned are covered in the closing section of the book.
The topic of image classification is presented as a thoroughly curated sequence of steps that gradually increase understanding of the working of a fully trainable classifier. Practical exercises in Python/Keras/Tensorflow have been designed to allow for experimental exploration of these concepts. In each chapter, suitable functions from Python modules are briefly introduced to provide students with the necessary tools to conduct these experiments.
More
Long description:
Image classification is a critical component in computer vision tasks and has numerous applications. Traditional methods for image classification involve feature extraction and classification in feature space. Current state-of-the-art methods utilize end-to-end learning with deep neural networks, where feature extraction and classification are integrated into the model. Understanding traditional image classification is important because many of its design concepts directly correspond to components of a neural network. This knowledge can help demystify the behavior of these networks, which may seem opaque at first sight.
The book starts from introducing methods for model-driven feature extraction and classification, including basic computer vision techniques for extracting high-level semantics from images. A brief overview of probabilistic classification with generative and discriminative classifiers is then provided. Next, neural networks are presented as a means to learn a classification model directly from labeled sample images, with individual components of the network discussed. The relationships between network components and those of a traditional designed model are explored, and different concepts for regularizing model training are explained. Finally, various methods for analyzing what a network has learned are covered in the closing section of the book.
The topic of image classification is presented as a thoroughly curated sequence of steps that gradually increase understanding of the working of a fully trainable classifier. Practical exercises in Python/Keras/Tensorflow have been designed to allow for experimental exploration of these concepts. In each chapter, suitable functions from Python modules are briefly introduced to provide students with the necessary tools to conduct these experiments.
More
Table of Contents:
Chapter 1. Image Classification ? A Computer Vision Task.- Chapter 2. Image Features ? Extraction and Categories.- Chapter 3. Feature Reduction.- Chapter 4. Bayesian Image Classification in Feature Space.- Chapter 5. Distance-based Classifiers.- Chapter 6. Decision Boundaries in Feature Space.- Chapter 7. Multi-layer Perceptron for Image Classification.- Chapter 8. Feature Extraction by Convolutional Neural Network.- Chapter 9. Network Set-up for Image Classification.- Chapter 10. Basic Network Training for Image Classification.- Chapter 11. Dealing with Training Deficiencies.- Chapter 12. Learning Effects and Network Decisions.
More
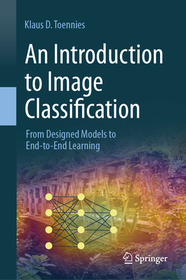
An Introduction to Image Classification: From Designed Models to End-to-End Learning
Subcribe now and receive a favourable price.
Subscribe
31 768 HUF